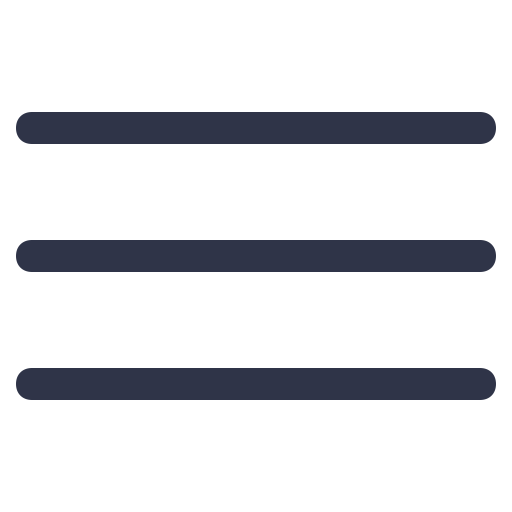
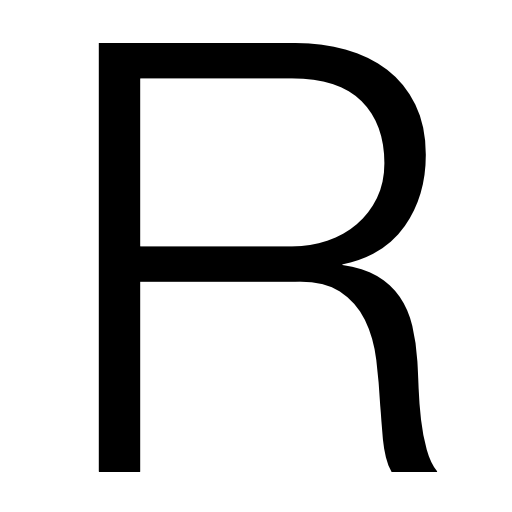
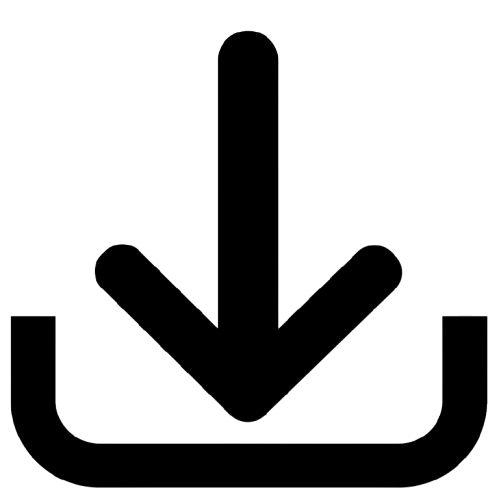
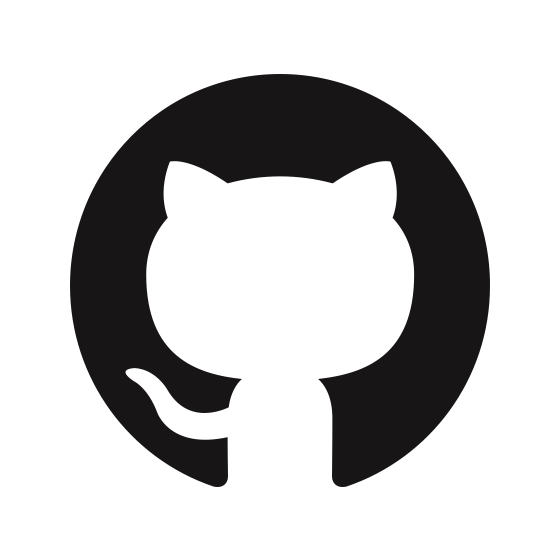
Bibliography
[1] Wikipedia contributors, Standard model — Wikipedia, the free encyclopedia, https://en.wikipedia.org/w/index.php?title=Standard_Model&oldid=1238968997 (2024), [Online; accessed 22-August-2024].
[2] ALEPH, DELPHI, L3, OPAL, SLD, LEP Electroweak Working Group, SLD Electroweak Group, SLD Heavy Flavour Group Collaboration, Precision electroweak measurements on the resonance, Phys. Rept. 427, 257–454 (2006), arXiv:hep-ex/0509008 .
[3] Wikipedia contributors, Yang–mills theory — Wikipedia, the free encyclopedia (2024), [Online; accessed 16-October-2024].
[4] Alex Dias and V. Pleitez, Grand Unification and Proton Stability Near the Peccei-Quinn Scale, Physical Review D 70 (2004).
[5] M. J. Duff and K. S. Stelle, Sir Thomas Walter Bannerman Kibble CBE, Biogr. Mems. Fell. R. Soc. 70, 225–244 (2021), arXiv:2011.13257 .
[6] Latham Boyle, Standard Model Of Particle Physics–Most Complete Diagram (2024), [Online; accessed 25-September-2024].
[7] Diogo Boito, Consequences of the renormalization group for perturbative quantum chromodynamics, Nature Phys. 19, 1533–1535 (2023).
[8] F. Bissey, F-G. Cao, A. R. Kitson, A. I. Signal, D. B. Leinweber, B. G. Lasscock, and A. G. Williams, Gluon flux-tube distribution and linear confinement in baryons, Phys. Rev. D 76, 114512 (2007), arXiv:hep-lat/0606016 .
[9] Derek B. Leinweber, Visualizations of Quantum Chromodynamics (2004), [Online; accessed 25-October-2024].
[10] Jun John Sakurai, Invariance Principles and Elementary Particles (Princeton University Press, 2015).
[11] Wikipedia contributors, Isospin — Wikipedia, the free encyclopedia (2024), [Online; accessed 26-October-2024].
[12] Wikipedia contributors, Deep inelastic scattering — Wikipedia, the free encyclopedia (2024), [Online; accessed 28-October-2024].
[13] ATLAS Collaboration, When protons collide (2024), [Online; accessed 28-October-2024].
[14] M. Krasny, F. Dydak, F. Fayette, W. Płaczek, and Andrzej Siodmok, at the LHC: a forlorn hope?, European Physical Journal C 69, 379–397 (2010).
[15] Charalampos Anastasiou, Lance J. Dixon, Kirill Melnikov, and Frank Petriello, High precision QCD at hadron colliders: Electroweak gauge boson rapidity distributions at NNLO, Phys. Rev. D 69, 094008 (2004), arXiv:hep-ph/0312266 .
[16] Monika Kollar, Top-Quark and Top-Squark Production at Hadron Colliders at Electroweak NLO (Technische Universitat Munchen, 2007).
[17] H1 Collaboration, Measurement of DIS cross section at HERA, Braz. J. Phys. 37, 793–797 (2007).
[18] Henning Kirschenmann, Jet Energy Scale Corrections and their Impact on Measurements of the Top-Quark Mass at CMS, Ph.D. thesis, U. Hamburg, Dept. Phys. (2014).
[19] L. A. T. Bauerdick et al., Event display for the visualization of CMS events, J. Phys. Conf. Ser. 331, 072039 (2011).
[20] CMS Collaboration, Search for highly energetic double Higgs boson production in the two bottom quark and two vector boson all-hadronic final state, CMS Physics Analysis Summary CMS-PAS-HIG-23-012 (2024).
[21] Gavin P. Salam, Elements of QCD for hadron colliders, in 2009 European School of High-Energy Physics (2010) arXiv:1011.5131 .
[22] Stefan Prestel, The Lund Hadronization Model (NuSTEC workshop, Gran Sasso Science Institute, 2018) [Online; accessed 29-October-2024].
[23] CMS Collaboration, A portrait of the Higgs boson by the CMS experiment ten years after the discovery., Nature 607, 60–68 (2022), [Erratum: Nature 623, (2023)], arXiv:2207.00043 .
[24] LHC Higgs Cross Section Working Group, Handbook of LHC Higgs Cross Sections: 2. Differential Distributions, CERN Yellow Reports: Monographs (CERN, Geneva, 2012).
[25] LHC Higgs Cross Section Working Group, Handbook of LHC Higgs Cross Sections: 4. Deciphering the Nature of the Higgs Sector, CERN Yellow Reports: Monographs (CERN, Geneva, 2017).
[26] CMS Collaboration, A measurement of the Higgs boson mass in the diphoton decay channel, Phys. Lett. B 805, 135425 (2020), arXiv:2002.06398 .
[27] Jason Veatch, Searches for Resonant Scalar Boson Pair Production Using Run 2 LHC Proton-Proton Collision Data, Symmetry 14, 260 (2022).
[28] CMS Collaboration, Search for Higgs Boson Pair Production in the Four b Quark Final State in Proton-Proton Collisions at s=13 TeV, Phys. Rev. Lett. 129, 081802 (2022), arXiv:2202.09617 .
[29] CMS Collaboration, Search for nonresonant Higgs boson pair production in final state with two bottom quarks and two tau leptons in proton-proton collisions at s=13 TeV, Phys. Lett. B 842, 137531 (2023), arXiv:2206.09401 .
[30] CMS Collaboration, Search for nonresonant Higgs boson pair production in final states with two bottom quarks and two photons in proton-proton collisions at = 13 TeV, JHEP 03, 257, arXiv:2011.12373 .
[31] CMS Collaboration, Search for Higgs boson pair production in the bbWW decay mode in proton-proton collisions at = 13 TeV, Submitted to JHEP (2024), arXiv:2403.09430 .
[32] CMS Collaboration, Combination of searches for nonresonant Higgs boson pair production in proton-proton collisions at , CMS Physics Analysis Summary CMS-PAS-HIG-20-011 (CERN, Geneva, 2024).
[33] Philippe Mouche, Overall view of the LHC. Vue d’ensemble du LHC (2014), general Photo.
[34] O. Bruning, H. Burkhardt, and S. Myers, The Large Hadron Collider, Prog. Part. Nucl. Phys. 67, 705–734 (2012).
[35] LHC Machine, JINST 3, S08001.
[36] CMS Collaboration, CMS Luminosity Public Results, https://twiki.cern.ch/twiki/bin/view/CMSPublic/LumiPublicResults (2024), [Online; accessed 13-November-2024].
[37] Rogelio Tomas Garcia, Luminosity forecasts, https://lhc-commissioning.web.cern.ch/schedule/hl-lhc-plots.htm (2024), [Online; accessed 10-November-2024].
[38] CERN, LHC / HL-LHC Plan, https://hilumilhc.web.cern.ch/content/hl-lhc-project (2024), [Online; accessed 3-December-2024].
[39] CMS Collaboration, Development of the CMS detector for the CERN LHC Run 3, JINST 19 (05), P05064, arXiv:2309.05466 .
[40] CMS Collaboration, Interactive Slice of the CMS detector (2016).
[41] Maximilien Brice and Claudia Marcelloni, Lowering the centre of CMS. Descente dans la caverne de la partie centrale du détecteur de particules CMS (Compact Muon Solenoid). (2007).
[42] CMS Collaboration, Precise Mapping of the Magnetic Field in the CMS Barrel Yoke using Cosmic Rays, JINST 5, T03021, arXiv:0910.5530 .
[43] CMS Collaboration, The Phase-2 Upgrade of the CMS Tracker, CMS Technical Design Report CERN-LHCC-2017-009, CMS-TDR-014 (2017).
[44] CMS Collaboration, Upgrade of the CMS Barrel Electromagnetic Calorimeter for the High Luminosity LHC, Instruments 6, 29 (2022).
[45] CMS Collaboration, CMS Technical Design Report for the Phase 1 Upgrade of the Hadron Calorimeter, CMS Technical Design Report CERN-LHCC-2012-015, CMS-TDR-010 (2012).
[46] CMS Collaboration, The CMS Experiment at the CERN LHC, JINST 3, S08004.
[47] CMS Collaboration, Performance of the CMS muon detector and muon reconstruction with proton-proton collisions at 13 TeV, JINST 13 (06), P06015, arXiv:1804.04528 .
[48] CMS Collaboration, Particle-flow reconstruction and global event description with the CMS detector, JINST 12, P10003, arXiv:1706.04965 .
[49] Thorben Quast, CMS Calorimeter Endcap Upgrade (HGCAL), in Beam Test Calorimeter Prototypes for the CMS Calorimeter Endcap Upgrade: Qualification, Performance Validation and Fast Generative Modelling (Springer International Publishing, 2021) pp. 45–62.
[50] Don Lincoln, ed., Instrumentation and Techniques in High Energy Physics (World Scientific, Singapore, 2024).
[51] Iuliana Tabian, Hailing Fu, and Zahra Sharif Khodaei, A Convolutional Neural Network for Impact Detection and Characterization of Complex Composite Structures, Sensors 19, 10.3390/s19224933 (2019).
[52] Robert D. Cousins, On Goodness–of–Fit Tests, in 2018 IEEE/CVF Conference on Computer Vision and Pattern Recognition (2016) pp. 849–858.
[53] Zhantao Chen, Nina Andrejevic, Tess Smidt, Zhiwei Ding, Qian Xu, Yen-Ting Chi, Quynh T. Nguyen, Ahmet Alatas, Jing Kong, and Mingda Li, Direct Prediction of Phonon Density of States With Euclidean Neural Networks, Advanced Science 8, 2004214 (2021).
[54] Alexander Bogatskiy, Brandon Anderson, Jan Offermann, Marwah Roussi, David Miller, and Risi Kondor, Lorentz group equivariant neural network for particle physics, in Proceedings of the 37th International Conference on Machine Learning, Proceedings of Machine Learning Research, Vol. 119 (PMLR, 2020) pp. 992–1002.
[55] Lilian Weng, From Autoencoder to Beta-VAE, lilianweng.github.io (2018).
[56] Lilian Weng, What are diffusion models?, lilianweng.github.io (2021).
[57] Matheus Gadelha, Subhransu Maji, and Rui Wang, Shape Generation using Spatially Partitioned Point Clouds, CoRR abs/1707.06267 (2017), arXiv:1707.06267 .
[58] Kyle Cranmer, Practical Statistics for the LHC, in 2011 European School of High-Energy Physics (2014) arXiv:1503.07622 .
[59] Caroline Stiller, Baseline assessment and effect of a supplementary community-based nutrition intervention study on the prevention/treatment of anemia among young Adivasi children in West Bengal, India, Ph.D. thesis, University of Hohenheim (2021).
[60] ATLAS Collaboration, Observation of a new particle in the search for the Standard Model Higgs boson with the ATLAS detector at the LHC, Phys. Lett. B 716, 1–29 (2012), arXiv:1207.7214 .
[61] Frédéric A. Dreyer, Gavin P. Salam, and Grégory Soyez, The Lund Jet Plane, JHEP 12, 064, arXiv:1807.04758 .
[62] CMS Collaboration, Lund Plane Reweighting for Jet Substructure Correction, CMS Detector Performance Note CMS-DP-2023-046 (2023).
[63] Arthur Gretton, GANs with integral probability metrics: some results and conjectures (2020), https://www.gatsby.ucl.ac.uk/ gretton/papers/oxford20.pdf.
[64] antike, What is the advantages of Wasserstein metric compared to Kullback-Leibler divergence?, https://stats.stackexchange.com/q/351153 (version: 2018-06-13).
[65] Wikipedia contributors, Type i and type ii errors — Wikipedia, the free encyclopedia (2024), [Online; accessed 7-December-2024].
[66] Raghav Kansal, Carlos Pareja, Zichun Hao, and Javier Duarte, JetNet: A Python Package for Accessing Open Datasets and Benchmarking Machine Learning Methods in High Energy Physics, J. Open Source Softw. 8, 5789 (2023).
[67] Steven Tsan, Raghav Kansal, Anthony Aportela, Daniel Diaz, Javier Duarte, Sukanya Krishna, Farouk Mokhtar, Jean-Roch Vlimant, and Maurizio Pierini, Particle Graph Autoencoders and Differentiable, Learned Energy Mover’s Distance, in 35th Conference on Neural Information Processing Systems (2021) arXiv:2111.12849 .
[68] Zichun Hao, Raghav Kansal, Javier Duarte, and Nadezda Chernyavskaya, Lorentz group equivariant autoencoders, Eur. Phys. J. C 83, 485 (2023), arXiv:2212.07347 .
[69] David E Morrissey and Michael J Ramsey-Musolf, Electroweak baryogenesis, New Journal of Physics 14, 125003 (2012).
[70] F. Bazzocchi and M. Fabbrichesi, Flavor and electroweak symmetry breaking at the TeV scale, Nuclear Physics B 715, 372–412 (2005).
[71] Spyros Argyropoulos, Oleg Brandt, and Ulrich Haisch, Collider Searches for Dark Matter through the Higgs Lens, Symmetry 13, 10.3390/sym13122406 (2021).
[72] Lawrence M. Krauss and James B. Dent, A Higgs–Saw Mechanism as a Source for Dark Energy, Phys. Rev. Lett. 111, 061802 (2013), arXiv:1306.3239 .
[73] H.R. Pagels, The Cosmic Code: Quantum Physics as the Language of Nature, Dover books on physics (Dover Publications, Incorporated, 2012) p. 295.
[74] David Tong, Lectures on Quantum Field Theory (University of Cambridge, 2019).
[75] David Tong, Lectures on Gauge Theory (University of Cambridge, 2018).
[76] David Tong, Lectures on the Standard Model (University of Cambridge, 2019).
[77] John McGreevy, Lectures notes on Symmetry in physics (University of California, San Diego, 2020).
[78] Frederic Schuller, Lectures on Geometrical Anatomy of Theoretical Physics (Friedrich-Alexander-Universität Erlangen-Nürnberg, 2016).
[79] Anthony Zee, Group Theory in a Nutshell for Physicists (Princeton University Press, USA, 2016).
[80] Anthony Zee, Quantum field theory in a nutshell (Princeton University Press, USA, 2003).
[81] Michael E. Peskin and Daniel V. Schroeder, An Introduction to quantum field theory (Addison-Wesley, Reading, USA, 1995).
[82] Hong Liu, Relativistic Quantum Field Theory (MIT OpenCourseWare, 2023).
[83] Ricardo D. Matheus, Quantum Field Theory II (Instituto de Física Teórica, 2023).
[84] Wikipedia contributors, Baker–campbell–hausdorff formula — Wikipedia, the free encyclopedia (2023), [Online; accessed 20-August-2024].
[85] C.G.J. Jacobi, Nova methodus, aequationes differentiales partiales primi ordinis inter numerum variabilium quemcunque propositas integrandi., Journal für die reine und angewandte Mathematik 60, 1–181 (1862).
[86] Matthew D. Schwartz, Quantum Field Theory and the Standard Model (Cambridge University Press, 2014).
[87] Eugene P. Wigner, On Unitary Representations of the Inhomogeneous Lorentz Group, Annals Math. 40, 149–204 (1939).
[88] user1379857 (https://physics.stackexchange.com/users/157704/user1379857), Proof that Lorentz group representation is a 4-vector, Physics Stack Exchange.
[89] Gerhart Lüders and Bruno Zumino, Connection between Spin and Statistics, Phys. Rev. 110, 1450–1453 (1958).
[90] Richard P. Feynman, Robert B. Leighton, and Matthew Sands, The Feynman Lectures on Physics, Vol. 3 (Addison-Wesley, 1965) p. 4.1.
[91] Robert Foot, H. Lew, X. G. He, and Girish C. Joshi, Seesaw Neutrino Masses Induced by a Triplet of Leptons, Z. Phys. C 44, 441 (1989).
[92] J. Schechter and J. W. F. Valle, Neutrino Masses in SU(2) x U(1) Theories, Phys. Rev. D 22, 2227 (1980).
[93] Werner Rodejohann, Neutrino-less Double Beta Decay and Particle Physics, Int. J. Mod. Phys. E 20, 1833–1930 (2011), arXiv:1106.1334 .
[94] CMS Collaboration, Search for heavy Majorana neutrinos in same-sign dilepton channels in proton-proton collisions at TeV, JHEP 01, 122, arXiv:1806.10905 .
[95] Steven Weinberg, The Search for Unity: Notes for a History of Quantum Field Theory, Daedalus 106, 17–35 (1977).
[96] Aneesh V. Manohar, Introduction to Effective Field Theories (Les Houches Summer School on Effective Field Theories, 2018) arXiv:1804.05863 .
[97] Gino Isidori, Felix Wilsch, and Daniel Wyler, The standard model effective field theory at work, Rev. Mod. Phys. 96, 015006 (2024), arXiv:2303.16922 .
[98] H. Lehmann, K. Symanzik, and W. Zimmermann, On the formulation of quantized field theories, Nuovo Cim. 1, 205–225 (1955).
[99] Hideki Yukawa, On the Interaction of Elementary Particles I, Proc. Phys. Math. Soc. Jap. 17, 48–57 (1935).
[100] C. M. G. Lattes, H. Muirhead, G. P. S. Occhialini, and C. F. Powell, Processes involving charged mesons, Nature 159, 694–697 (1947).
[101] Zhong-Zhi Xianyu, A Complete Solution to Problems in “An Introduction to Quantum Field Theory” by Peskin and Schroeder (2016), [Online; accessed 29-August-2024].
[102] Dan-di Wu, A Brief Introduction to the Strong CP Problem, Z. Naturforsch. A 52, 179–181 (1997).
[103] Thomas Mannel, Theory and phenomenology of CP violation, Nucl. Phys. B Proc. Suppl. 167, 115–119 (2007).
[104] Chen-Ning Yang and Robert L. Mills, Conservation of Isotopic Spin and Isotopic Gauge Invariance, Phys. Rev. 96, 191–195 (1954).
[105] H. David Politzer, Reliable Perturbative Results for Strong Interactions?, Phys. Rev. Lett. 30, 1346–1349 (1973).
[106] David J. Gross and Frank Wilczek, Ultraviolet Behavior of Nonabelian Gauge Theories, Phys. Rev. Lett. 30, 1343–1346 (1973).
[107] Philip W. Anderson, Plasmons, Gauge Invariance, and Mass, Phys. Rev. 130, 439–442 (1963).
[108] F. Englert and R. Brout, Broken Symmetry and the Mass of Gauge Vector Mesons, Phys. Rev. Lett. 13, 321–323 (1964).
[109] Peter W. Higgs, Broken Symmetries and the Masses of Gauge Bosons, Phys. Rev. Lett. 13, 508–509 (1964).
[110] G. S. Guralnik, C. R. Hagen, and T. W. B. Kibble, Global Conservation Laws and Massless Particles, Phys. Rev. Lett. 13, 585–587 (1964).
[111] Yoichiro Nambu and G. Jona-Lasinio, Dynamical Model of Elementary Particles Based on an Analogy with Superconductivity. 1., Phys. Rev. 122, 345–358 (1961).
[112] Yoichiro Nambu and G. Jona-Lasinio, Dynamical model of elementary particles based on an analogy with superconductivity. II., Phys. Rev. 124, 246–254 (1961).
[113] Sheldon L. Glashow, The renormalizability of vector meson interactions, Nucl. Phys. 10, 107–117 (1959).
[114] Abdus Salam, Weak and Electromagnetic Interactions, Conf. Proc. C 680519, 367–377 (1968).
[115] Steven Weinberg, A Model of Leptons, Phys. Rev. Lett. 19, 1264–1266 (1967).
[116] S. Elitzur, Impossibility of Spontaneously Breaking Local Symmetries, Phys. Rev. D 12, 3978–3982 (1975).
[117] Werner Heisenberg, Über den bau der atomkerne. i, Zeitschrift für Physik 77, 1–11 (1932).
[118] Eugene P. Wigner, On the Consequences of the Symmetry of the Nuclear Hamiltonian on the Spectroscopy of Nuclei, Physical Review 51, 106–119 (1937).
[119] Murray Gell-Mann, The Eightfold Way: A Theory of strong interaction symmetry (1961).
[120] Yuval Ne’eman, Derivation of strong interactions from a gauge invariance, Nucl. Phys. 26, 222–229 (1961).
[121] Murray Gell-Mann, A Schematic Model of Baryons and Mesons, Phys. Lett. 8, 214–215 (1964).
[122] George Zweig, An SU(3) model for strong interaction symmetry and its breaking. 1., Phys. Rev. Lett. 12, 132–135 (1964).
[123] S. L. Glashow, J. Iliopoulos, and L. Maiani, Weak Interactions with Lepton-Hadron Symmetry, Phys. Rev. D 2, 1285–1292 (1970).
[124] SLAC-SP-017 Collaboration, Discovery of a Narrow Resonance in Annihilation, Phys. Rev. Lett. 33, 1406–1408 (1974).
[125] E598 Collaboration, Experimental Observation of a Heavy Particle , Phys. Rev. Lett. 33, 1404–1406 (1974).
[126] Makoto Kobayashi and Toshihide Maskawa, CP Violation in the Renormalizable Theory of Weak Interaction, Prog. Theor. Phys. 49, 652–657 (1973).
[127] Martin L. Perl et al., Evidence for Anomalous Lepton Production in e+ - e- Annihilation, Phys. Rev. Lett. 35, 1489–1492 (1975).
[128] E288 Collaboration, Observation of a Dimuon Resonance at 9.5-GeV in 400-GeV Proton-Nucleus Collisions, Phys. Rev. Lett. 39, 252–255 (1977).
[129] CDF Collaboration, Observation of top quark production in collisions, Phys. Rev. Lett. 74, 2626–2631 (1995), arXiv:hep-ex/9503002 .
[130] D0 Collaboration, Observation of the top quark, Phys. Rev. Lett. 74, 2632–2637 (1995), arXiv:hep-ex/9503003 .
[131] John C. Collins, Davison E. Soper, and George F. Sterman, Factorization of Hard Processes in QCD, Adv. Ser. Direct. High Energy Phys. 5, 1–91 (1989), arXiv:hep-ph/0409313 .
[132] Torsten Pfoh, LHC Theory Lectures (2012), [Online; accessed 29-October-2024].
[133] Joey Huston, Introduction to QCD from an LHC perspective (2018), [Online; accessed 29-October-2024].
[134] J. Alwall, R. Frederix, S. Frixione, V. Hirschi, F. Maltoni, O. Mattelaer, H. S. Shao, T. Stelzer, P. Torrielli, and M. Zaro, The automated computation of tree-level and next-to-leading order differential cross sections, and their matching to parton shower simulations, JHEP 07, 079, arXiv:1405.0301 .
[135] Katharina Danziger, Stefan Höche, and Frank Siegert, Reducing negative weights in Monte Carlo event generation with Sherpa, arXiv:2110.15211 (2021).
[136] Torbjörn Sjöstrand, Stefan Ask, Jesper R. Christiansen, Richard Corke, Nishita Desai, Philip Ilten, Stephen Mrenna, Stefan Prestel, Christine O. Rasmussen, and Peter Z. Skands, An introduction to PYTHIA 8.2, Comput. Phys. Commun. 191, 159–177 (2015), arXiv:1410.3012 .
[137] G. Corcella, I. G. Knowles, G. Marchesini, S. Moretti, K. Odagiri, P. Richardson, M. H. Seymour, and B. R. Webber, HERWIG 6: An Event generator for hadron emission reactions with interfering gluons (including supersymmetric processes), JHEP 01, 010, arXiv:hep-ph/0011363 .
[138] Sherpa Collaboration, Event Generation with Sherpa 2.2, SciPost Phys. 7, 034 (2019), arXiv:1905.09127 .
[139] Johan Alwall et al., Comparative study of various algorithms for the merging of parton showers and matrix elements in hadronic collisions, Eur. Phys. J. C 53, 473–500 (2008), arXiv:0706.2569 .
[140] Stefan Höche, Introduction to parton-shower event generators, in Theoretical Advanced Study Institute in Elementary Particle Physics: Journeys Through the Precision Frontier: Amplitudes for Colliders (2015) pp. 235–295, arXiv:1411.4085 .
[141] Stefan Höche, Introduction to Parton Showers (School and Workshop on pQCD, West Lake, 2018) [Online; accessed 29-October-2024].
[142] Bo Andersson, G. Gustafson, G. Ingelman, and T. Sjostrand, Parton Fragmentation and String Dynamics, Phys. Rept. 97, 31–145 (1983).
[143] E. Fermi, An attempt of a theory of beta radiation. 1., Z. Phys. 88, 161–177 (1934).
[144] Gargamelle Neutrino Collaboration, Observation of Neutrino Like Interactions Without Muon Or Electron in the Gargamelle Neutrino Experiment, Phys. Lett. B 46, 138–140 (1973).
[145] UA1 Collaboration, Experimental Observation of Isolated Large Transverse Energy Electrons with Associated Missing Energy at GeV, Phys. Lett. B 122, 103–116 (1983).
[146] UA1 Collaboration, Experimental Observation of Lepton Pairs of Invariant Mass Around 95-GeV/c**2 at the CERN SPS Collider, Phys. Lett. B 126, 398–410 (1983).
[147] UA2 Collaboration, Observation of Single Isolated Electrons of High Transverse Momentum in Events with Missing Transverse Energy at the CERN anti-p p Collider, Phys. Lett. B 122, 476–485 (1983).
[148] UA2 Collaboration, Evidence for at the CERN Collider, Phys. Lett. B 129, 130–140 (1983).
[149] CMS Collaboration, Observation of a New Boson at a Mass of 125 GeV with the CMS Experiment at the LHC, Phys. Lett. B 716, 30–61 (2012), arXiv:1207.7235 .
[150] Paul A. M. Dirac, New basis for cosmology, Proc. Roy. Soc. Lond. A 165, 199–208 (1938).
[151] M. Gell-Mann, The interpretation of the new particles as displaced charge multiplets, Nuovo Cim. 4, 848–866 (1956).
[152] J. Wess and J. Bagger, Supersymmetry and supergravity (Princeton University Press, Princeton, NJ, USA, 1992).
[153] Nathaniel Craig, Jamison Galloway, and Scott Thomas, Searching for Signs of the Second Higgs Doublet, arXiv:1305.2424 (2013).
[154] Tania Robens, Tim Stefaniak, and Jonas Wittbrodt, Two-real-scalar-singlet extension of the SM: LHC phenomenology and benchmark scenarios, Eur. Phys. J. C 80, 151 (2020), arXiv:1908.08554 .
[155] Nathaniel Craig, Naturalness and New Approaches to the Hierarchy Problem (Prospects in Theoretical Physics, Institute for Advanced Study, 2017) [Online; accessed 30-October-2024].
[156] Ziro Maki, Masami Nakagawa, and Shoichi Sakata, Remarks on the unified model of elementary particles, Prog. Theor. Phys. 28, 870–880 (1962).
[157] LHC Higgs Cross Section Working Group Collaboration, Handbook of LHC Higgs Cross Sections: 3. Higgs Properties (2013) arXiv:1307.1347 .
[158] Manuel Reichert, Astrid Eichhorn, Holger Gies, Jan M. Pawlowski, Tilman Plehn, and Michael M. Scherer, Probing baryogenesis through the Higgs boson self-coupling, Phys. Rev. D 97, 075008 (2018), arXiv:1711.00019 .
[159] Massimiliano Grazzini, Gudrun Heinrich, Stephen Jones, Stefan Kallweit, Matthias Kerner, Jonas M. Lindert, and Javier Mazzitelli, Higgs boson pair production at NNLO with top quark mass effects, JHEP 05, 059, arXiv:1803.02463 .
[160] J. Baglio, F. Campanario, S. Glaus, M. Mühlleitner, J. Ronca, and M. Spira, : Combined uncertainties, Phys. Rev. D 103, 056002 (2021), arXiv:2008.11626 .
[161] Fady Bishara, Roberto Contino, and Juan Rojo, Higgs pair production in vector-boson fusion at the LHC and beyond, Eur. Phys. J. C 77, 481 (2017), arXiv:1611.03860 .
[162] CMS Collaboration, Search for Nonresonant Pair Production of Highly Energetic Higgs Bosons Decaying to Bottom Quarks, Phys. Rev. Lett. 131, 041803 (2023), arXiv:2205.06667 .
[163] ATLAS Collaboration, Search for pair production of boosted Higgs bosons via vector-boson fusion production in the final state using collisions at TeV with the ATLAS detector, ATLAS Conference Note ATLAS-CONF-2024-003 (2024).
[164] CMS Collaboration, Performance of heavy-flavour jet identification in boosted topologies in proton-proton collisions at , CMS Physics Analysis Summary CMS-PAS-BTV-22-001 (2023).
[165] G. C. Branco, P. M. Ferreira, L. Lavoura, M. N. Rebelo, Marc Sher, and Joao P. Silva, Theory and phenomenology of two-Higgs-doublet models, Phys. Rept. 516, 1–102 (2012), arXiv:1106.0034 .
[166] Florian Domingo and Sebastian Paßehr, About the bosonic decays of heavy Higgs states in the (N)MSSM, Eur. Phys. J. C 82, 962 (2022), arXiv:2207.05776 .
[167] CMS Collaboration, Search for a massive scalar resonance decaying to a light scalar and a Higgs boson in the four b quarks final state with boosted topology, Phys. Lett. B 842, 137392 (2023), arXiv:2204.12413 .
[168] CMS Collaboration, Search for a new resonance decaying into two spin-0 bosons in a final state with two photons and two bottom quarks in proton-proton collisions at = 13 TeV, Submitted to JHEP (2023), arXiv:2310.01643 .
[169] CMS Collaboration, Search for a heavy Higgs boson decaying into two lighter Higgs bosons in the bb final state at 13 TeV, JHEP 11, 057, arXiv:2106.10361 .
[170] Bernhard Wolf, Handbook of Ion Sources (CRC Press, Boca Raton, FL, 1995).
[171] J. P. Blewett, 200-GeV Intersecting Storage Accelerators, eConf C710920, 501 (1971).
[172] Oliver Brüning and Lucio Rossi, eds., The High Luminosity Large Hadron Collider: The new machine for illuminating the mysteries of Universe, Vol. 24 (World Scientific, 2015).
[173] CMS Collaboration, Performance of the CMS Level-1 trigger in proton-proton collisions at TeV, JINST 15, P10017, arXiv:2006.10165 .
[174] CMS Collaboration, The CMS trigger system, JINST 12 (01), P01020, arXiv:1609.02366 .
[175] CMS Collaboration, A CMS Level-1 Track Finder for the HL-LHC, Tech. Rep. (2021) arXiv:2110.02826 .
[176] Werner Riegler, Particle Physics Instrumentation, https://indico.cern.ch/event/208901/contributions/1501073/attachments/323336/450953/Riegler_L1.pdf (2013), [Online; accessed 12-November-2024].
[177] Hans A. Bethe and Julius Ashkin, Passage of Radiations Through Matter, in Experimental Nuclear Physics, Vol. 1 (John Wiley & Sons, New York, 1953) pp. 166–357.
[178] LHCb Collaboration, The LHCb Detector at the LHC, JINST 3, S08005.
[179] John Sparkes, Semiconductor Devices, 2nd ed. (CRC Press, Boca Raton, FL, 1994) p. 78.
[180] Andrei Nomerotski, Silicon detectors for tracking and vertexing, Nucl. Instrum. Meth. A 598, 33–40 (2009).
[181] RD50 Collaboration, Radiation hard semiconductor devices for very high luminosity colliders, PoS VERTEX2015, 032 (2015).
[182] CMS Collaboration, Description and performance of track and primary-vertex reconstruction with the CMS tracker, JINST 9 (10), P10009, arXiv:1405.6569 .
[183] CMS Collaboration, Reconstruction of signal amplitudes in the CMS electromagnetic calorimeter in the presence of overlapping proton-proton interactions, JINST 15 (10), P10002, arXiv:2006.14359 .
[184] CMS Collaboration, The CMS-HF quartz fiber calorimeters, J. Phys. Conf. Ser. 160, 012014 (2009).
[185] CMS Collaboration, The Performance of the CMS Muon Detector in Proton-Proton Collisions at = 7 TeV at the LHC, JINST 8, P11002, arXiv:1306.6905 .
[186] CMS Collaboration, The Phase-2 Upgrade of the CMS Muon Detectors, CMS Technical Design Report CERN-LHCC-2017-012, CMS-TDR-016 (2017).
[187] R. Fruhwirth, Application of Kalman filtering to track and vertex fitting, Nucl. Instrum. Meth. A 262, 444–450 (1987).
[188] CMS Collaboration, Identification of b quark jets at the CMS Experiment in the LHC Run 2, CMS Physics Analysis Summary CMS-PAS-BTV-15-001 (2016).
[189] CMS Collaboration, Electron and photon reconstruction and identification with the CMS experiment at the CERN LHC, JINST 16 (05), P05014, arXiv:2012.06888 .
[190] CMS Collaboration, Performance of the local reconstruction algorithms for the CMS hadron calorimeter with Run 2 data, JINST 18 (11), P11017, arXiv:2306.10355 .
[191] CMS Collaboration, Calibration of the CMS hadron calorimeters using proton-proton collision data at 13 TeV, JINST 15 (05), P05002, arXiv:1910.00079 .
[192] CMS Collaboration, Technical proposal for the Phase-II upgrade of the Compact Muon Solenoid, CMS Technical Proposal CERN-LHCC-2015-010, CMS-TDR-15-02 (2015).
[193] Matteo Cacciari, Gavin P. Salam, and Gregory Soyez, The anti- jet clustering algorithm, JHEP 04, 063, arXiv:0802.1189 .
[194] Matteo Cacciari, Gavin P. Salam, and Gregory Soyez, FastJet user manual, Eur. Phys. J. C 72, 1896 (2012), arXiv:1111.6097 .
[195] CMS Collaboration, Pileup Removal Algorithms, CMS Physics Analysis Summary CMS-PAS-JME-14-001 (2014).
[196] Daniele Bertolini, Philip Harris, Matthew Low, and Nhan Tran, Pileup Per Particle Identification, JHEP 10, 059, arXiv:1407.6013 .
[197] CMS Collaboration, Pileup mitigation at CMS in 13 TeV data, JINST 15, P09018, arXiv:2003.00503 .
[198] CMS Collaboration, Performance of Electron Reconstruction and Selection with the CMS Detector in Proton-Proton Collisions at 8 TeV, JINST 10 (06), P06005, arXiv:1502.02701 .
[199] C. Foudas, A. Rose, J. Jones, and G. Hall, A Study for a Tracking Trigger at First Level for CMS at SLHC (2005), arXiv:physics/0510227 .
[200] Aidan D. Chambers, Neural-Network Based b-Tagging in the CMS Level 1 Trigger System, Bachelor’s thesis, Massachusetts Institute of Technology (2023).
[201] CMS Collaboration, CMS timing detectors in Phase-2, PoS LHCP2019, 250 (2019).
[202] CMS Collaboration, Physics opportunities with a MIP Timing Detector in CMS at HL-LHC, CMS Conference Report CMS-CR-2022-199 (CERN, Geneva, 2023).
[203] CMS Collaboration, A MIP Timing Detector for the CMS Phase-2 Upgrade, CMS Technical Design Report CERN-LHCC-2019-003, CMS-TDR-020 (2019).
[204] CMS Collaboration, The Phase-2 Upgrade of the CMS Barrel Calorimeters, CMS Technical Design Report CERN-LHCC-2017-011, CMS-TDR-015 (CERN, Geneva, 2017) this is the final version, approved by the LHCC.
[205] CMS Collaboration, The Phase-2 Upgrade of the CMS Endcap Calorimeter, CMS Technical Design Report CERN-LHCC-2017-023, CMS-TDR-019 (2017).
[206] CMS HGCAL Collaboration, Timing Performance of the CMS High Granularity Calorimeter Prototype, JINST 19 (04), P04015, arXiv:2312.14622 .
[207] CMS Collaboration, Operation of CMS GE1/1 GEM detectors in Run-3, CMS Conference Report CMS-CR-2024-210 (CERN, Geneva, 2024).
[208] CMS Muon Collaboration, The upgrade of the CMS muon system for the high luminosity LHC, JINST 19 (02), C02077, arXiv:2312.05939 .
[209] Trevor Hastie, Robert Tibshirani, and Jerome H. Friedman, Boosting and Additive Trees, in The Elements of Statistical Learning (Springer, New York, 2009) pp. 337–384, 2nd ed.
[210] HEP ML Community, A Living Review of Machine Learning for Particle Physics.
[211] Corinna Cortes and Vladimir Vapnik, Support-Vector Networks, Machine Learning 20, 273–297 (1995).
[212] Leo Breiman, Jerome H. Friedman, Richard A. Olshen, and Charles J. Stone, Classification and Regression Trees (Wadsworth International Group, 1984).
[213] Olivier Chapelle, Bernhard Schölkopf, and Alexander Zien, eds., Semi-Supervised Learning (MIT Press, Cambridge, MA, 2006).
[214] Randall Balestriero, Mark Ibrahim, Vlad Sobal, Ari Morcos, Shashank Shekhar, Tom Goldstein, Florian Bordes, Adrien Bardes, Gregoire Mialon, Yuandong Tian, Avi Schwarzschild, Andrew Gordon Wilson, Jonas Geiping, Quentin Garrido, Pierre Fernandez, Amir Bar, Hamed Pirsiavash, Yann LeCun, and Micah Goldblum, A Cookbook of Self-Supervised Learning (2023), arXiv:2304.12210 .
[215] Geoffrey Hinton, Neural Networks for Machine Learning – Lecture 6e – RMSProp, Coursera Lecture Slides (2012), https://www.cs.toronto.edu/~tijmen/csc321/slides/lecture_slides_lec6.pdf.
[216] Diederik P. Kingma and Jimmy Ba, Adam: A Method for Stochastic Optimization, in Proceedings of the 3rd International Conference on Learning Representations (ICLR) (2015).
[217] Lutz Prechelt, Early Stopping—But When?, in Neural Networks: Tricks of the Trade, Lecture Notes in Computer Science, Vol. 7700 (Springer, Berlin, Heidelberg, 2012) pp. 53–67.
[218] Nitish Srivastava, Geoffrey Hinton, Alex Krizhevsky, Ilya Sutskever, and Ruslan Salakhutdinov, Dropout: A Simple Way to Prevent Neural Networks from Overfitting, Journal of Machine Learning Research 15, 1929–1958 (2014).
[219] Sergey Ioffe and Christian Szegedy, Batch Normalization: Accelerating Deep Network Training by Reducing Internal Covariate Shift, in Proceedings of the 32nd International Conference on Machine Learning, Proceedings of Machine Learning Research, Vol. 37 (PMLR, Lille, France, 2015) pp. 448–456.
[220] Frank Rosenblatt, The Perceptron: A Probabilistic Model for Information Storage and Organization in the Brain, Psychological Review 65, 386–408 (1958).
[221] David E. Rumelhart, Geoffrey E. Hinton, and Ronald J. Williams, Learning Representations by Back-Propagating Errors, Nature 323, 533–536 (1986).
[222] Kurt Hornik, Maxwell Stinchcombe, and Halbert White, Multilayer Feedforward Networks are Universal Approximators, Neural Networks 2, 359–366 (1989).
[223] Alex Krizhevsky, Ilya Sutskever, and Geoffrey E. Hinton, ImageNet Classification with Deep Convolutional Neural Networks, in Advances in Neural Information Processing Systems, Vol. 25 (2012) pp. 1097–1105.
[224] Kaiming He, Xiangyu Zhang, Shaoqing Ren, and Jian Sun, Deep Residual Learning for Image Recognition, in Proceedings of the IEEE Conference on Computer Vision and Pattern Recognition (2016) pp. 770–778.
[225] Ashish Vaswani, Noam Shazeer, Niki Parmar, Jakob Uszkoreit, Llion Jones, Aidan N. Gomez, Lukasz Kaiser, and Illia Polosukhin, Attention Is All You Need, in Advances in Neural Information Processing Systems, Vol. 30 (2017) pp. 5998–6008.
[226] Tom B. Brown, Benjamin Mann, Nick Ryder, Melanie Subbiah, Jared Kaplan, Prafulla Dhariwal, Arvind Neelakantan, Pranav Shyam, Girish Sastry, Amanda Askell, Sandhini Agarwal, Ariel Herbert-Voss, Gretchen Krueger, Tom Henighan, Rewon Child, Aditya Ramesh, Daniel M. Ziegler, Jeffrey Wu, Clemens Winter, Christopher Hesse, Mark Chen, Eric Sigler, Mateusz Litwin, Scott Gray, Benjamin Chess, Jack Clark, Christopher Berner, Sam McCandlish, Alec Radford, Ilya Sutskever, and Dario Amodei, Language Models are Few-Shot Learners, Advances in Neural Information Processing Systems 33, 1877–1901 (2020).
[227] Luke de Oliveira, Michael Kagan, Lester Mackey, Benjamin Nachman, and Ariel Schwartzman, Jet-images — deep learning edition, JHEP 07, 069, arXiv:1511.05190 .
[228] CMS Collaboration, Identification of heavy, energetic, hadronically decaying particles using machine-learning techniques, JINST 15 (06), P06005, arXiv:2004.08262 .
[229] ATLAS Collaboration, Deep generative models for fast shower simulation in ATLAS, Tech. Rep. ATL-SOFT-PUB-2018-001 (CERN, Geneva, 2018).
[230] Huilin Qu and Loukas Gouskos, ParticleNet: Jet Tagging via Particle Clouds, Phys. Rev. D 101, 056019 (2020), arXiv:1902.08570 .
[231] Nathaniel Thomas, Tess Smidt, Steven Kearnes, Lusann Yang, Li Li, Kai Kohlhoff, and Patrick Riley, Tensor field networks: Rotation- and translation-equivariant neural networks for 3D point clouds, arXiv:1802.08219 (2018).
[232] Taco Cohen and Max Welling, Group Equivariant Convolutional Networks, in Proceedings of The 33rd International Conference on Machine Learning, Proceedings of Machine Learning Research, Vol. 48 (PMLR, New York, New York, USA, 2016) pp. 2990–2999.
[233] Taco S. Cohen and Max Welling, Steerable CNNs, in International Conference on Learning Representations (2017).
[234] D. E. Worrall, S. J. Garbin, D. Turmukhambetov, and G. J. Brostow, Harmonic Networks: Deep Translation and Rotation Equivariance, in 2017 IEEE Conference on Computer Vision and Pattern Recognition (CVPR) (IEEE Computer Society, Los Alamitos, CA, USA, 2017) pp. 7168–7177.
[235] Maurice Weiler and Gabriele Cesa, General E(2)-Equivariant Steerable CNNs, in Advances in Neural Information Processing Systems, Vol. 32 (Curran Associates, Inc., 2019).
[236] Maurice Weiler, Mario Geiger, Max Welling, Wouter Boomsma, and Taco S Cohen, 3D Steerable CNNs: Learning Rotationally Equivariant Features in Volumetric Data, in Advances in Neural Information Processing Systems, Vol. 31 (Curran Associates, Inc., 2018).
[237] Benjamin Kurt Miller, Mario Geiger, Tess E. Smidt, and Frank Noé, Relevance of Rotationally Equivariant Convolutions for Predicting Molecular Properties (2020) arXiv:2008.08461 .
[238] Stephan Eismann, Raphael J.L. Townshend, Nathaniel Thomas, Milind Jagota, Bowen Jing, and Ron O. Dror, Hierarchical, rotation-equivariant neural networks to select structural models of protein complexes, Proteins: Structure, Function, and Bioinformatics 89, 493–501 (2021), arXiv:https://onlinelibrary.wiley.com/doi/pdf/10.1002/prot.26033 .
[239] Risi Kondor, Zhen Lin, and Shubhendu Trivedi, Clebsch-Gordan Nets: a fully Fourier space spherical convolutional neural network (2018).
[240] Brandon Anderson, Truong-Son Hy, and Risi Kondor, Cormorant: Covariant Molecular Neural Networks (2019).
[241] Maurice Weiler and Gabriele Cesa, General E(2)-equivariant steerable CNNs, in Advances in Neural Information Processing Systems, Vol. 32 (Curran Associates, Inc., 2019).
[242] I.M. Gelfand, R.A. Minlos, and Z.Y. Shapiro, Representations of the Rotation and Lorentz Groups and Their Applications (Dover Publications, 2018).
[243] Taco S Cohen, Mario Geiger, and Maurice Weiler, A General Theory of Equivariant CNNs on Homogeneous Spaces, in Advances in Neural Information Processing Systems, Vol. 32 (Curran Associates, Inc., 2019).
[244] Marc Finzi, Samuel Stanton, Pavel Izmailov, and Andrew Gordon Wilson, Generalizing convolutional neural networks for equivariance to lie groups on arbitrary continuous data, in 37th International Conference on Machine Learning (2020) pp. 3146–3157, arXiv:2002.12880 .
[245] G. E. Hinton and R. R. Salakhutdinov, Reducing the Dimensionality of Data with Neural Networks, Science 313, 504 (2006).
[246] Giuseppe Di Guglielmo et al., A Reconfigurable Neural Network ASIC for Detector Front-End Data Compression at the HL-LHC, IEEE Trans. Nucl. Sci. 68, 2179 (2021), arXiv:2105.01683 .
[247] Theo Heimel, Gregor Kasieczka, Tilman Plehn, and Jennifer M. Thompson, QCD or What?, SciPost Phys. 6, 030 (2019), arXiv:1808.08979 .
[248] Marco Farina, Yuichiro Nakai, and David Shih, Searching for New Physics with Deep Autoencoders, Phys. Rev. D 101, 075021 (2020), arXiv:1808.08992 .
[249] Olmo Cerri, Thong Q. Nguyen, Maurizio Pierini, Maria Spiropulu, and Jean-Roch Vlimant, Variational Autoencoders for New Physics Mining at the Large Hadron Collider, JHEP 05, 036, arXiv:1811.10276 .
[250] Thorben Finke, Michael Krämer, Alessandro Morandini, Alexander Mück, and Ivan Oleksiyuk, Autoencoders for unsupervised anomaly detection in high energy physics, JHEP 06, 161, arXiv:2104.09051 .
[251] Gregor Kasieczka et al., The LHC Olympics 2020 a community challenge for anomaly detection in high energy physics, Rept. Prog. Phys. 84, 124201 (2021), arXiv:2101.08320 .
[252] Ekaterina Govorkova et al., Autoencoders on field-programmable gate arrays for real-time, unsupervised new physics detection at 40 MHz at the Large Hadron Collider, Nat. Mach. Intell. 4, 154 (2022), arXiv:2108.03986 .
[253] Adrian Alan Pol, Victor Berger, Gianluca Cerminara, Cecile Germain, and Maurizio Pierini, Anomaly Detection With Conditional Variational Autoencoders, in 18th International Conference on Machine Learning and Applications (2020) arXiv:2010.05531 .
[254] Vishal S. Ngairangbam, Michael Spannowsky, and Michihisa Takeuchi, Anomaly detection in high-energy physics using a quantum autoencoder, Phys. Rev. D 105, 095004 (2022), arXiv:2112.04958 .
[255] Barry M. Dillon, Gregor Kasieczka, Hans Olischlager, Tilman Plehn, Peter Sorrenson, and Lorenz Vogel, Symmetries, safety, and self-supervision, SciPost Phys. 12, 188 (2022), arXiv:2108.04253 .
[256] CMS Collaboration, Model-agnostic search for dijet resonances with anomalous jet substructure in proton-proton collisions at = 13 TeV, CMS Physics Analysis Summary CMS-PAS-EXO-22-026 (CERN, Geneva, 2024).
[257] CMS Collaboration, Anomaly Detection in the CMS Global Trigger Test Crate for Run 3, CMS Detector Performance Note CMS-DP-2023-079 (2023).
[258] CMS Collaboration, 2024 Data Collected with AXOL1TL Anomaly Detection at the CMS Level-1 Trigger, CMS Detector Performance Note CMS-DP-2024-059 (2024).
[259] Dor Bank, Noam Koenigstein, and Raja Giryes, Autoencoders, arXiv:2003.05991 (2020).
[260] Michael Tschannen, Olivier Bachem, and Mario Lucic, Recent Advances in Autoencoder-Based Representation Learning, arXiv:1812.05069 (2018).
[261] Diederik P Kingma and Max Welling, Auto-Encoding Variational Bayes, in 2nd International Conference on Learning Representations, ICLR, Conference Track Proceedings (2014) arXiv:1312.6114 .
[262] Oliver Atkinson, Akanksha Bhardwaj, Christoph Englert, Vishal S. Ngairangbam, and Michael Spannowsky, Anomaly detection with convolutional Graph Neural Networks, JHEP 08, 080, arXiv:2105.07988 .
[263] Laurent Dinh, Jascha Sohl-Dickstein, and Samy Bengio, Density estimation using Real NVP, in International Conference on Learning Representations (2017).
[264] Diederik P. Kingma and Prafulla Dhariwal, Glow: Generative Flow with Invertible Convolutions, in Advances in Neural Information Processing Systems, Vol. 31 (2018) pp. 10215–10224.
[265] Claudius Krause and David Shih, Fast and accurate simulations of calorimeter showers with normalizing flows, Phys. Rev. D 107, 113003 (2023), arXiv:2106.05285 .
[266] Tor Fjelde, Emile Mathieu, and Vincent Dutordoir, An Introduction to Flow Matching (2024), online; accessed 2024-11-26.
[267] Scott H. Hawley, Flow With What You Know (2024), online; accessed 2024-11-26.
[268] Xingchao Liu, Chengyue Gong, and qiang liu, Flow Straight and Fast: Learning to Generate and Transfer Data with Rectified Flow, in The Eleventh International Conference on Learning Representations (2023).
[269] Sangyun Lee, Zinan Lin, and Giulia Fanti, Improving the Training of Rectified Flows, in The Thirty-eighth Annual Conference on Neural Information Processing Systems (2024).
[270] Breno Orzari, Nadezda Chernyavskaya, Raphael Cobe, Javier Duarte, Jefferson Fialho, Dimitrios Gunopulos, Raghav Kansal, Maurizio Pierini, Thiago Tomei, and Mary Touranakou, LHC hadronic jet generation using convolutional variational autoencoders with normalizing flows, Machine Learning: Science and Technology 4, 045023 (2023).
[271] Ian J. Goodfellow, Jean Pouget-Abadie, Mirza Mehdi, Bing Xu, David Warde-Farley, Ozair Sherjil, Aaron Courville, and Yoshua Bengio, Generative Adversarial Nets, in Advances in Neural Information Processing Systems 27 (Curran Associates, Inc., 2014) p. 2672, arXiv:1406.2661 .
[272] Martin Arjovsky, Soumith Chintala, and Léon Bottou, Wasserstein generative adversarial networks, in Proceedings of the 34th International Conference on Machine Learning, Proceedings of Machine Learning Research, Vol. 70 (PMLR, 2017) pp. 214–223.
[273] Tim Salimans, Ian Goodfellow, Wojciech Zaremba, Vicki Cheung, Alec Radford, and Xi Chen, Improved techniques for training GANs, in Advances in Neural Information Processing Systems, Vol. 29 (2016).
[274] Martin Arjovsky and Léon Bottou, Towards Principled Methods for Training Generative Adversarial Networks, in International Conference on Learning Representations (2017).
[275] Lars Mescheder, Andreas Geiger, and Sebastian Nowozin, Which training methods for GANs do actually converge?, in Proceedings of the 35th International Conference on Machine Learning, Vol. 80 (2018) pp. 3481–3490.
[276] Tero Karras, Samuli Laine, and Timo Aila, A Style-Based Generator Architecture for Generative Adversarial Networks, in Proceedings of the IEEE/CVF Conference on Computer Vision and Pattern Recognition (CVPR) (2019) pp. 4401–4410.
[277] Andrew Brock, Jeff Donahue, and Karen Simonyan, Large scale GAN training for high fidelity natural image synthesis, in International Conference on Learning Representations (ICLR) (2019).
[278] Tero Karras, Samuli Laine, Miika Aittala, Janne Hellsten, Jaakko Lehtinen, and Timo Aila, Analyzing and improving the image quality of StyleGAN, in Proceedings of the IEEE/CVF Conference on Computer Vision and Pattern Recognition (CVPR) (2020) pp. 8110–8119.
[279] Luke de Oliveira, Michela Paganini, and Benjamin Nachman, Learning Particle Physics by Example: Location-Aware Generative Adversarial Networks for Physics Synthesis, Comput. Softw. Big Sci. 1, 4 (2017), arXiv:1701.05927 .
[280] Michela Paganini, Luke de Oliveira, and Benjamin Nachman, CaloGAN : Simulating 3D high energy particle showers in multilayer electromagnetic calorimeters with generative adversarial networks, Phys. Rev. D 97, 014021 (2018), arXiv:1712.10321 .
[281] ATLAS Collaboration, Fast simulation of the ATLAS calorimeter system with Generative Adversarial Networks, ATLAS Software Public Note ATL-SOFT-PUB-2020-006 (2020).
[282] Martin Erdmann, Jonas Glombitza, and Thorben Quast, Precise simulation of electromagnetic calorimeter showers using a Wasserstein generative adversarial network, Comput. Softw. Big Sci. 3, 4 (2019), arXiv:1807.01954 .
[283] Federico Carminati, Gulrukh Khattak, Vladimir Loncar, Thong Q. Nguyen, Maurizio Pierini, Ricardo Brito Da Rocha, Konstantinos Samaras-Tsakiris, Sofia Vallecorsa, and Jean-Roch Vlimant, Generative Adversarial Networks for fast simulation, J. Phys. Conf. Ser. 1525, 012064 (2020).
[284] Jonathan Ho, Ajay Jain, and Pieter Abbeel, Denoising Diffusion Probabilistic Models, in Advances in Neural Information Processing Systems, Vol. 33 (2020) pp. 6840–6851.
[285] Yang Song, Jascha Sohl-Dickstein, Diederik P. Kingma, Abhishek Kumar, Stefano Ermon, and Ben Poole, Score-Based Generative Modeling through Stochastic Differential Equations, in International Conference on Learning Representations (2021).
[286] Prafulla Dhariwal and Alex Nichol, Diffusion models beat GANs on image synthesis, in Advances in Neural Information Processing Systems, Vol. 34 (2021) pp. 8780–8794.
[287] Yadong Lu, Julian Collado, Daniel Whiteson, and Pierre Baldi, Sparse autoregressive models for scalable generation of sparse images in particle physics, Phys. Rev. D 103, 036012 (2021), arXiv:2009.14017 .
[288] Angel X. Chang, Thomas Funkhouser, Leonidas Guibas, Pat Hanrahan, Qixing Huang, Zimo Li, Silvio Savarese, Manolis Savva, Shuran Song, Hao Su, Jianxiong Xiao, Li Yi, and Fisher Yu, ShapeNet: An Information-Rich 3D Model Repository, Technical Report (2015) arXiv:1512.03012 .
[289] Chun-Liang Li, Manzil Zaheer, Yang Zhang, Barnabas Poczos, and Ruslan Salakhutdinov, Point cloud GAN, in 2019 ICLR Workshop Deep Generative Models for Highly Structured Data (2018) arXiv:1810.05795 .
[290] Guandao Yang, Xun Huang, Zekun Hao, Ming-Yu Liu, Serge Belongie, and Bharath Hariharan, PointFlow: 3D point cloud generation with continuous normalizing flows, in 2019 IEEE/CVF International Conference on Computer Vision (ICCV) (2019) p. 4540, arXiv:1906.12320 .
[291] Roman Klokov, Edmond Boyer, and Jakob Verbeek, Discrete Point Flow Networks for Efficient Point Cloud Generation, in 2020 European Conference on Computer Vision (ECCV) (Springer International Publishing, Cham, 2020) p. 694, arXiv:2007.10170 .
[292] Ruojin Cai, Guandao Yang, Hadar Averbuch-Elor, Zekun Hao, Serge Belongie, Noah Snavely, and Bharath Hariharan, Learning Gradient Fields for Shape Generation, in 2020 European Conference on Computer Vision (ECCV) (Cham, 2020) p. 364, arXiv:2008.06520 .
[293] Jonas Köhler, Leon Klein, and Frank Noe, Equivariant Flows: Exact Likelihood Generative Learning for Symmetric Densities, in Proceedings of the 37th International Conference on Machine Learning, Proceedings of Machine Learning Research, Vol. 119 (PMLR, 2020) p. 5361, arXiv:2006.02425 .
[294] Gregor N. C. Simm, Robert Pinsler, Gábor Csányi, and José Miguel Hernández-Lobato, Symmetry-Aware Actor-Critic for 3D Molecular Design, in International Conference on Learning Representations (2021).
[295] Niklas Gebauer, Michael Gastegger, and Kristof Schütt, Symmetry-adapted generation of 3D point sets for the targeted discovery of molecules, in Advances in Neural Information Processing Systems 32 (Curran Associates, Inc., 2019) p. 7566, arXiv:1906.00957 .
[296] Panos Achlioptas, Olga Diamanti, Ioannis Mitliagkas, and Leonidas Guibas, Learning representations and generative models for 3D point clouds, in Proceedings of the 35th International Conference on Machine Learning, Vol. 80 (PMLR, 2018) p. 40.
[297] Diego Valsesia, Giulia Fracastoro, and Enrico Magli, Learning localized generative models for 3D point clouds via graph convolution, in International Conference on Learning Representations (ICLR) 2019 (2019).
[298] Dong Wook Shu, Sung Woo Park, and Junseok Kwon, 3D point cloud generative adversarial network based on tree structured graph convolutions, in 2019 IEEE/CVF International Conference on Computer Vision (ICCV) (2019) p. 3858, arXiv:1905.06292 .
[299] Charles R. Qi, Hao Su, Kaichun Mo, and Leonidas J. Guibas, PointNet: Deep learning on point sets for 3D classification and segmentation, in Proceedings of the IEEE Conference on Computer Vision and Pattern Recognition (CVPR) (2017).
[300] He Wang, Zetian Jiang, Li Yi, Kaichun Mo, Hao Su, and Leonidas J Guibas, Rethinking sampling in 3D point cloud generative adversarial networks, arXiv:2006.07029 (2020).
[301] Glen Cowan, Kyle Cranmer, Eilam Gross, and Ofer Vitells, Asymptotic formulae for likelihood-based tests of new physics, Eur. Phys. J. C 71, 1554 (2011), [Erratum: Eur.Phys.J.C 73, 2501 (2013)], arXiv:1007.1727 .
[302] Charles R. Harris et al., Array programming with NumPy, Nature 585, 357–362 (2020).
[303] Pauli Virtanen et al., SciPy 1.0: Fundamental Algorithms for Scientific Computing in Python, Nature Methods 17, 261–272 (2020).
[304] J. D. Hunter, Matplotlib: A 2D graphics environment, Computing in Science & Engineering 9, 90–95 (2007).
[305] Wikipedia contributors, Informant (statistics) — Wikipedia, the free encyclopedia (2024), [Online; accessed 7-December-2024].
[306] Wikipedia contributors, Central limit theorem — Wikipedia, the free encyclopedia, https://en.wikipedia.org/w/index.php?title=Central_limit_theorem&oldid=1257009135 (2024), [Online; accessed 7-December-2024].
[307] Wikipedia contributors, Law of large numbers — Wikipedia, the free encyclopedia, https://en.wikipedia.org/w/index.php?title=Law_of_large_numbers&oldid=1256071749 (2024), [Online; accessed 7-December-2024].
[308] Gregory Gundersen, Asymptotic Normality of MLE, https://gregorygundersen.com/blog/2019/11/28/asymptotic-normality-mle/ (2019), [Online; accessed 7-December-2024].
[309] Wikipedia contributors, Noncentral chi-squared distribution — Wikipedia, the free encyclopedia (2024), [Online; accessed 7-December-2024].
[310] GEANT4 Collaboration, GEANT4: A Simulation toolkit, Nucl. Instrum. Methods Phys. Res. A A506, 250 (2003).
[311] CMS Collaboration, Current and future performance of the CMS simulation, EPJ Web Conf. 214, 02036 (2019).
[312] CMS Offline Software and Computing, CMS Phase-2 Computing Model: Update Document, CMS Note CMS-NOTE-2022-008 (2022) https://cds.cern.ch/record/2815292.
[313] Torbjörn Sjöstrand, Stefan Ask, Christiansen Jesper R., Richard Corke, Nishita Desai, Ilten Philip, Stephen Mrenna, Stefan Prestel, Christine O. Rasmussen, and Peter Z. Skands, An introduction to pythia 8.2, Comput. Phys. Commun. 191, 159 (2015), arXiv:1410.3012 .
[314] Johannes Bellm et al., herwig 7.0/herwig ++3.0 release note, Eur. Phys. J. C 76, 196 (2016), arXiv:1512.01178 .
[315] Maurizio Pierini, Javier Mauricio Duarte, Nhan Tran, and Marat Freytsis, hls4ml LHC jet dataset (150 particles) (2020).
[316] Johan Alwall, Michel Herquet, Fabio Maltoni, Olivier Mattelaer, and Tim Stelzer, MadGraph 5 : Going Beyond, JHEP 06, 128, arXiv:1106.0522 .
[317] Richard D. Ball et al., Parton distributions with LHC data, Nucl. Phys. B 867, 244–289 (2013), arXiv:1207.1303 .
[318] Peter Skands, Stefano Carrazza, and Juan Rojo, Tuning pythia 8.1: The Monash 2013 Tune, Eur. Phys. J. C 74, 3024 (2014), arXiv:1404.5630 .
[319] Matteo Cacciari, Gavin P. Salam, and Gregory Soyez, FastJet user manual, Eur. Phys. J. C 72, 1896 (2012), arXiv:1111.6097 .
[320] M. Cacciari and G. P. Salam, Dispelling the myth for the jet-finder, Phys. Lett. B 641, 57 (2006), arXiv:hep-ph/0512210 .
[321] CMS Collaboration, Recent Developments in CMS Fast Simulation, PoS ICHEP2016, 181 (2016), arXiv:1701.03850 .
[322] Patrick T. Komiske, Eric M. Metodiev, and Jesse Thaler, Energy flow polynomials: A complete linear basis for jet substructure, JHEP 04, 013, arXiv:1712.07124 .
[323] Ali Borji, Pros and cons of GAN evaluation measures: New developments, arXiv:2103.09396 (2021).
[324] Martin Heusel, Hubert Ramsauer, Thomas Unterthiner, Bernhard Nessler, and Sepp Hochreiter, GANs trained by a two time-scale update rule converge to a local nash equilibrium, in Advances in Neural Information Processing Systems, Vol. 30 (Curran Associates, Inc., Red Hook, NY, 2017) p. 6626, arXiv:1706.08500 .
[325] Patrick T. Komiske, Eric M. Metodiev, and Jesse Thaler, Metric Space of Collider Events, Phys. Rev. Lett. 123, 041801 (2019), arXiv:1902.02346 .
[326] Andrew J. Larkoski, Ian Moult, and Benjamin Nachman, Jet Substructure at the Large Hadron Collider: A Review of Recent Advances in Theory and Machine Learning, Phys. Rept. 841, 1 (2020), arXiv:1709.04464 .
[327] Yue Wang, Yongbin Sun, Ziwei Liu, Sanjay E. Sarma, Michael M. Bronstein, and Justin M. Solomon, Dynamic graph CNN for learning on point clouds, ACM Trans. Graph. 38, 10.1145/3326362 (2019), arXiv:1801.07829 .
[328] Federico Monti, Davide Boscaini, Jonathan Masci, Emanuele Rodolà, Jan Svoboda, and Michael M. Bronstein, Geometric deep learning on graphs and manifolds using mixture model CNNs, in 2017 IEEE Conference on Computer Vision and Pattern Recognition (CVPR) (IEEE, New York, NY, 2017) p. 5425, arXiv:1611.08402 .
[329] Li Deng, The mnist database of handwritten digit images for machine learning research, IEEE Signal Processing Magazine 29, 141–142 (2012).
[330] Raghav Kansal, JetNet Library v0.0.3 (2021).
[331] Raghav Kansal, MPGAN v1.0.0 (2021).
[332] Cheng Chen, Olmo Cerri, Thong Q. Nguyen, Jean-Roch Vlimant, and Maurizio Pierini, Data augmentation at the LHC through analysis-specific fast simulation with deep learning, arXiv:2010.01835 (2020).
[333] Karl Stelzner, Kristian Kersting, and Adam R Kosiorek, Generative adversarial set transformers, in Workshop on Object-Oriented Learning at ICML, Vol. 3 (2020) p. 1.
[334] Juho Lee, Yoonho Lee, Jungtaek Kim, Adam R. Kosiorek, Seungjin Choi, and Yee Whye Teh, Set Transformer: A Framework for Attention-based Permutation-Invariant Neural Networks, in Proceedings of the 36th International Conference on Machine Learning, Vol. 97 (2019) p. 3744, arXiv:1810.00825 .
[335] Xudong Mao, Qing Li, Haoran Xie, Raymond Y. K. Lau, and Zhen Wang, Multi-class generative adversarial networks with the L2 loss function, arXiv:1611.04076 (2016).
[336] Raghav Kansal, Javier Duarte, Carlos Pareja, and Zichun Hao, jet-net/JetNet: v0.2.3 (2023).
[337] https://www.physics.ucla.edu/~cousins/ stats/ongoodness6march2016.pdf.
[338] Simon Badger et al., Machine Learning and LHC Event Generation, in 2022 Snowmass Summer Study (2022) arXiv:2203.07460 .
[339] Bharath K. Sriperumbudur, Kenji Fukumizu, Arthur Gretton, Bernhard Schölkopf, and Gert R. G. Lanckriet, On the empirical estimation of integral probability metrics, Electron. J. Stat. 6, 1550 (2012).
[340] Alfred Müller, Integral Probability Metrics and Their Generating Classes of Functions, Adv. Appl. Probab. 29, 429 (1997).
[341] L. V. Kantorovich, Mathematical Methods of Organizing and Planning Production, Manage. Sci. 6, 366 (1960).
[342] C. Villani, Optimal Transport: Old and New, Grundlehren der mathematischen Wissenschaften, Vol. 338 (Springer Berlin, 2008).
[343] Arthur Gretton, Karsten M. Borgwardt, Malte J. Rasch, Bernhard Schölkopf, and Alexander Smola, A Kernel Two-Sample Test, J. Mach. Learn. Res. 13, 723 (2012).
[344] S. Kullback and R. A. Leibler, On Information and Sufficiency, Ann. Stat. 22, 79 (1951).
[345] J. Lin, Divergence measures based on the Shannon entropy, IEEE Trans Inf. Theory 37, 145 (1991).
[346] Andrew K. C. Wong and Manlai You, Entropy and Distance of Random Graphs with Application to Structural Pattern Recognition, IEEE Trans. Pattern Anal. Mach. Intell. 7, 599 (1985).
[347] Karl Pearson, On the criterion that a given system of deviations from the probable in the case of a correlated system of variables is such that it can be reasonably supposed to have arisen from random sampling, Lond. Edinb. Dublin Philos. Mag. J. Sci. 50, 157 (1900).
[348] Steve Baker and Robert D. Cousins, Clarification of the Use of Chi Square and Likelihood Functions in Fits to Histograms, Nucl. Instrum. Meth. 221, 437 (1984).
[349] Robert D. Cousins, Generalization of Chisquare Goodness-of-Fit Test for Binned Data Using Saturated Models, with Application to Histograms (2013), http://www.physics.ucla.edu/~cousins/stats/cousins_saturated.pdf.
[350] J. K. Lindsey, Parametric Statistical Inference (Clarendon Press, 1996).
[351] Carl-Johann Simon-Gabriel, Alessandro Barp, and Lester W. Mackey, Metrizing Weak Convergence with Maximum Mean Discrepancies, arXiv:2006.09268 (2020).
[352] Bharath K. Sriperumbudur, Kenji Fukumizu, Arthur Gretton, Bernhard Schölkopf, and Gert R. G. Lanckriet, On integral probability metrics, -divergences and binary classification, arXiv:0901.2698 .
[353] R.J. Vanderbei, Linear Programming: Foundations and Extensions, International Series in Operations Research & Management Science (Springer US, 2013).
[354] Aaditya Ramdas, Nicolás García Trillos, and Marco Cuturi, On Wasserstein Two-Sample Testing and Related Families of Nonparametric Tests, Entropy 19, 47 (2017).
[355] Christian Szegedy, Vincent Vanhoucke, Sergey Ioffe, Jon Shlens, and Zbigniew Wojna, Rethinking the Inception Architecture for Computer Vision, in 2016 IEEE Conference on Computer Vision and Pattern Recognition (CVPR) (2016) p. 2818, arXiv:1512.00567 .
[356] Mikołaj Bińkowski, Dougal J. Sutherland, Michael Arbel, and Arthur Gretton, Demystifying MMD GANs, in International Conference on Learning Representations (2018).
[357] Min Jin Chong and David Forsyth, Effectively unbiased FID and Inception score and where to find them, in Proceedings of the IEEE/CVF Conference on Computer Vision and Pattern Recognition (CVPR) (2020) arXiv:1911.07023 .
[358] Arthur Gretton, Karsten Borgwardt, Malte Rasch, Bernhard Schölkopf, and Alex Smola, A Kernel Method for the Two-Sample-Problem, in Advances in Neural Information Processing Systems, Vol. 19 (MIT Press, 2006) arXiv:0805.2368 .
[359] Feng Liu, Wenkai Xu, Jie Lu, Guangquan Zhang, Arthur Gretton, and Danica J. Sutherland, Learning Deep Kernels for Non-Parametric Two-Sample Tests, in Proceedings of the 37th International Conference on Machine Learning, Proceedings of Machine Learning Research, Vol. 119 (PMLR, 2020) pp. 6316–6326.
[360] Bharath K. Sriperumbudur, Arthur Gretton, Kenji Fukumizu, Bernhard Schölkopf, and Gert R.G. Lanckriet, Hilbert Space Embeddings and Metrics on Probability Measures, J. Mach. Learn. Res. 11, 1517 (2010).
[361] Tuomas Kynkäänniemi, Tero Karras, Samuli Laine, Jaakko Lehtinen, and Timo Aila, Improved Precision and Recall Metric for Assessing Generative Models, in Advances in Neural Information Processing Systems, Vol. 32 (Curran Associates, Inc., Red Hook, NY, 2019) arXiv:1904.06991 .
[362] Muhammad Ferjad Naeem, Seong Joon Oh, Youngjung Uh, Yunjey Choi, and Jaejun Yoo, Reliable Fidelity and Diversity Metrics for Generative Models, in Proceedings of the 37th International Conference on Machine Learning, Proceedings of Machine Learning Research, Vol. 119 (PMLR, 2020) pp. 7176–7185.
[363] Jerome H. Friedman, On multivariate goodness of fit and two sample testing, in PHYSTAT 2003, Vol. C030908 (SLAC, Stanford, CA, 2003) p. THPD002.
[364] David Lopez-Paz and Maxime Oquab, Revisiting Classifier Two-Sample Tests, in International Conference on Learning Representations (2017).
[365] Claudius Krause and David Shih, CaloFlow: Fast and Accurate Generation of Calorimeter Showers with Normalizing Flows, arXiv:2106.05285 (2021).
[366] Fast Calorimeter Simulation Challenge 2022, https://calochallenge.github.io/homepage/ (2022).
[367] Riccardo Guidotti, Anna Monreale, Salvatore Ruggieri, Franco Turini, Fosca Giannotti, and Dino Pedreschi, A Survey of Methods for Explaining Black Box Models, ACM Comput. Surv. 51, 10.1145/3236009 (2018), arXiv:1802.01933 .
[368] Raffaele Tito D’Agnolo and Andrea Wulzer, Learning New Physics from a Machine, Phys. Rev. D 99, 015014 (2019), arXiv:1806.02350 .
[369] Raffaele Tito D’Agnolo, Gaia Grosso, Pierini Maurizio, Andrea Wulzer, and Marco Zanetti, Learning Multivariate New Physics, Eur. Phys. J. C 81, 89 (2021), arXiv:1912.12155 .
[370] Simone Marzani, Gregory Soyez, and Michael Spannowsky, Looking inside jets: an introduction to jet substructure and boosted-object phenomenology, 1st ed., Lecture Notes in Physics, Vol. 958 (Springer, Cham, 2019) arXiv:1901.10342 .
[371] Stephanie Baffioni, Claude Charlot, Ferri Federico, David Futyan, Paolo Meridiani, Puljak Ivica, Chiara Rovelli, Roberto Salerno, and Sirois Yves, Electron reconstruction in CMS, CMS Note CMS-NOTE-2006-040 (2006) http://cds.cern.ch/record/934070.
[372] ATLAS Collaboration, Expected photon performance in the ATLAS experiment, ATLAS Note ATL-PHYS-PUB-2011-007 (2011) https://cds.cern.ch/record/1345329.
[373] D. Bertsimas and J.N. Tsitsiklis, Introduction to linear optimization (Athena Scientific, Nashua, NH, 1997).
[374] Rémi Flamary et al., POT: Python Optimal Transport, J. Mach. Learn. Res. 22, 1 (2021).
[375] Benno Käch, Dirk Krücker, Isabell Melzer-Pellmann, Moritz Scham, Simon Schnake, and Alexi Verney-Provatas, JetFlow: Generating Jets with Conditioned and Mass Constrained Normalising Flows, arXiv:2211.13630 (2022).
[376] Benno Käch, Dirk Krücker, and Isabell Melzer-Pellmann, Point Cloud Generation using Transformer Encoders and Normalising Flows, arXiv:2211.13623 (2022).
[377] Erik Buhmann, Gregor Kasieczka, and Jesse Thaler, EPiC-GAN: Equivariant Point Cloud Generation for Particle Jets, SciPost Phys. 15, 130 (2023), arXiv:2301.08128 .
[378] Matthew Leigh, Debajyoti Sengupta, Guillaume Quétant, John Andrew Raine, Knut Zoch, and Tobias Golling, PC-JeDi: Diffusion for particle cloud generation in high energy physics, SciPost Phys. 16, 018 (2024), arXiv:2303.05376 .
[379] Vinicius Mikuni, Benjamin Nachman, and Mariel Pettee, Fast point cloud generation with diffusion models in high energy physics, Phys. Rev. D 108, 036025 (2023), arXiv:2304.01266 .
[380] Benno Kach and Isabell Melzer-Pellmann, Attention to Mean-Fields for Particle Cloud Generation, arXiv:2305.15254 (2023).
[381] Matthew Leigh, Debajyoti Sengupta, John Andrew Raine, Guillaume Quétant, and Tobias Golling, Faster diffusion model with improved quality for particle cloud generation, Phys. Rev. D 109, 012010 (2024), arXiv:2307.06836 .
[382] Moritz A. W. Scham, Dirk Krücker, Benno Käch, and Kerstin Borras, DeepTreeGAN: Fast Generation of High Dimensional Point Clouds, EPJ Web Conf. 295, 09010 (2024), arXiv:2311.12616 .
[383] Moritz Alfons Wilhelm Scham, Dirk Krücker, and Kerstin Borras, DeepTreeGANv2: Iterative Pooling of Point Clouds, arXiv:2312.00042 (2023).
[384] Erik Buhmann, Cedric Ewen, Darius A. Faroughy, Tobias Golling, Gregor Kasieczka, Matthew Leigh, Guillaume Quétant, John Andrew Raine, Debajyoti Sengupta, and David Shih, EPiC-ly Fast Particle Cloud Generation with Flow-Matching and Diffusion, arXiv:2310.00049 (2023).
[385] Cheng Jiang, Sitian Qian, and Huilin Qu, Choose Your Diffusion: Efficient and flexible ways to accelerate the diffusion model in fast high energy physics simulation, arXiv:2401.13162 (2024).
[386] Cheng Jiang, Sitian Qian, and Huilin Qu, BUFF: Boosted Decision Tree based Ultra-Fast Flow matching, arXiv:2404.18219 (2024).
[387] Vinicius Mikuni and Benjamin Nachman, OmniLearn: A Method to Simultaneously Facilitate All Jet Physics Tasks, arXiv:2404.16091 (2024).
[388] Yi-An Chen and Kai-Feng Chen, Jet Discrimination with Quantum Complete Graph Neural Network, arXiv:2403.04990 (2024).
[389] Claudius Krause et al., CaloChallenge 2022: A Community Challenge for Fast Calorimeter Simulation, arXiv:2410.21611 (2024).
[390] Jack Y. Araz, Vinicius Mikuni, Felix Ringer, Nobuo Sato, Fernando Torales Acosta, and Richard Whitehill, Point cloud-based diffusion models for the Electron-Ion Collider, arXiv:2410.22421 (2024).
[391] Huilin Qu, Congqiao Li, and Sitian Qian, Particle Transformer for Jet Tagging, in Proceedings of the 39th International Conference on Machine Learning (2022) pp. 18281–18292, arXiv:2202.03772 .
[392] CMS Collaboration, Search for resonances decaying to three W bosons in proton-proton collisions at = 13 TeV, Phys. Rev. Lett. 129, 021802 (2022), arXiv:2201.08476 .
[393] CMS Collaboration, Identification of highly Lorentz-boosted heavy particles using graph neural networks and new mass decorrelation techniques, CMS Detector Performance Note CMS-DP-2020-002 (2020).
[394] Andrew J. Larkoski, Simone Marzani, Soyez Gregory, and Jesse Thaler, Soft Drop, JHEP 05, 146, arXiv:1402.2657 .
[395] S. Catani, Yuri L. Dokshitzer, M. H. Seymour, and B. R. Webber, Longitudinally invariant clustering algorithms for hadron hadron collisions, Nucl. Phys. B 406, 187–224 (1993).
[396] Stephen D. Ellis and Davison E. Soper, Successive combination jet algorithm for hadron collisions, Phys. Rev. D 48, 3160–3166 (1993), arXiv:hep-ph/9305266 .
[397] CMS Collaboration, CMS High Level Trigger Performance for Run 3, PoS EPS-HEP2023, 517 (2024).
[398] CMS Collaboration, Jet energy scale and resolution measurement with Run 2 Legacy Data Collected by CMS at 13 TeV, Tech. Rep. (2021).
[399] CMS Collaboration, Search for Higgs boson pair production via vector boson fusion with highly Lorentz-boosted Higgs bosons in the four b quark final state at TeV, CMS Physics Analysis Summary CMS-PAS-B2G-21-001 (CERN, 2021).
[400] S. Mrenna and P. Skands, Automated Parton-Shower Variations in Pythia 8, Phys. Rev. D 94, 074005 (2016), arXiv:1605.08352 .
[401] CMS Collaboration, Precision luminosity measurement in proton-proton collisions at 13 TeV in 2015 and 2016 at CMS, Eur. Phys. J. C 81, 800 (2021), arXiv:2104.01927 .
[402] CMS Collaboration, CMS luminosity measurement for the 2017 data-taking period at = 13 TeV, CMS Physics Analysis Summary CMS-PAS-LUM-17-004 (2018).
[403] CMS Collaboration, CMS luminosity measurement for the 2018 data-taking period at = 13 TeV, CMS Physics Analysis Summary CMS-PAS-LUM-18-002 (2019).
[404] Yifan Chen et al., A FAIR and AI-ready Higgs boson decay dataset, Sci. Data 9, 31 (2022), arXiv:2108.02214 .
[405] Adam Paszke, Sam Gross, Francisco Massa, Adam Lerer, James Bradbury, Gregory Chanan, Trevor Killeen, Zeming Lin, Natalia Gimelshein, Luca Antiga, Alban Desmaison, Andreas Kopf, Edward Yang, Zachary DeVito, Martin Raison, Alykhan Tejani, Sasank Chilamkurthy, Benoit Steiner, Lu Fang, Junjie Bai, and Soumith Chintala, PyTorch: An Imperative Style, High-Performance Deep Learning Library, in Advances in Neural Information Processing Systems, Vol. 32 (Curran Associates, Inc., 2019) p. 8024, arXiv:1912.01703 .
[406] Patrick T. Komiske, Radha Mastandrea, Eric M. Metodiev, Preksha Naik, and Jesse Thaler, Exploring the Space of Jets with CMS Open Data, Phys. Rev. D 101, 034009 (2020), arXiv:1908.08542 .
[407] Gregor Kasieczka, Tilman Plehn, Jennifer Thompson, and Michael Russel, Top Quark Tagging Reference Dataset (2019).
[408] Anja Butter et al., The Machine Learning landscape of top taggers, SciPost Phys. 7, 014 (2019), arXiv:1902.09914 .
[409] Patrick Komiske, Eric Metodiev, and Jesse Thaler, Pythia8 Quark and Gluon Jets for Energy Flow (2019).
[410] European Organization For Nuclear Research and OpenAIRE, Zenodo (2013).
[411] Raghav Kansal, Javier Duarte, Hao Su, Breno Orzari, Thiago Tomei, Maurizio Pierini, Mary Touranakou, Jean-Roch Vlimant, and Dimitrios Gunopulos, Particle Cloud Generation with Message Passing Generative Adversarial Networks, in Advances in Neural Information Processing Systems, Vol. 34 (Curran Associates, Inc., 2021) arXiv:2106.11535 .
[412] Raghav Kansal, Anni Li, Javier Duarte, Nadezda Chernyavskaya, Maurizio Pierini, Breno Orzari, and Thiago Tomei, Evaluating generative models in high energy physics, Phys. Rev. D 107, 076017 (2023), arXiv:2211.10295 .
[413] James Bradbury, Roy Frostig, Peter Hawkins, Matthew James Johnson, Chris Leary, Dougal Maclaurin, George Necula, Adam Paszke, Jake VanderPlas, Skye Wanderman-Milne, and Qiao Zhang, JAX: composable transformations of Python+NumPy programs (2018).
[414] Jack H. Collins, An Exploration of Learnt Representations of W Jets, in ICLR workshop Deep Generative Models for Highly Structured Data (2021) arXiv:2109.10919 .
[415] J. W. Monk, Deep Learning as a Parton Shower, JHEP 12, 021, arXiv:1807.03685 .
[416] Florencia Canelli, Annapaola de Cosa, Luc Le Pottier, Jeremi Niedziela, Kevin Pedro, and Maurizio Pierini, Autoencoders for semivisible jet detection, JHEP 02, 074, arXiv:2112.02864 .
[417] Taoli Cheng, Jean-François Arguin, Julien Leissner-Martin, Jacinthe Pilette, and Tobias Golling, Variational Autoencoders for Anomalous Jet Tagging, Phys. Rev. D (2020), arXiv:2007.01850 .
[418] Blaž Bortolato, Aleks Smolkovič, Barry M. Dillon, and Jernej F. Kamenik, Bump hunting in latent space, Phys. Rev. D 105, 115009 (2022), arXiv:2103.06595 .
[419] Sang Eon Park, Dylan Rankin, Silviu-Marian Udrescu, Mikaeel Yunus, and Philip Harris, Quasi Anomalous Knowledge: Searching for new physics with embedded knowledge, JHEP 21, 030, arXiv:2011.03550 .
[420] Shiqi Gong, Qi Meng, Jue Zhang, Huilin Qu, Congqiao Li, Sitian Qian, Weitao Du, Zhi-Ming Ma, and Tie-Yan Liu, An efficient Lorentz equivariant graph neural network for jet tagging, JHEP 07, 030, arXiv:2201.08187 .
[421] Congqiao Li, Huilin Qu, Sitian Qian, Qi Meng, Shiqi Gong, Jue Zhang, Tie-Yan Liu, and Qiang Li, Does Lorentz-symmetric design boost network performance in jet physics?, arXiv:2208.07814 (2022).
[422] Anja Butter, Gregor Kasieczka, Tilman Plehn, and Michael Russell, Deep-learned Top Tagging with a Lorentz Layer, SciPost Phys. 5, 028 (2018), arXiv:1707.08966 .
[423] Justin Gilmer, Samuel S. Schoenholz, Patrick F. Riley, Oriol Vinyals, and George E. Dahl, Neural Message Passing for Quantum Chemistry, in Proceedings of the 34th International Conference on Machine Learning, Vol. 70 (PMLR, 2017) p. 1263, arXiv:1704.01212 .
[424] Adam Paszke et al., PyTorch: An imperative style, high-performance deep learning library, in Advances in Neural Information Processing Systems, Vol. 32 (Curran Associates, Inc., 2019) p. 8024, arXiv:1912.01703 .
[425] Zichun Hao, Raghav Kansal, Javier Duarte, and Nadezda Chernyavskaya, Lorentz Group Equivariance Autoencoders (2022).
[426] R. Jonker and A. Volgenant, A shortest augmenting path algorithm for dense and sparse linear assignment problems, Computing 38, 325 (1987).
[427] Giulia Vilone and Luca Longo, Explainable Artificial Intelligence: a Systematic Review (2020), arXiv:2006.00093 .
[428] Dang Minh, H. Xiang Wang, Y. Fen Li, and Tan N. Nguyen, Explainable Artificial Intelligence: A Comprehensive Review, Artif. Intell. Rev. 55, 3503–3568 (2022).
[429] R. J. Adler, B. Casey, and O. C. Jacob, Vacuum catastrophe: An Elementary exposition of the cosmological constant problem, Am. J. Phys. 63, 620–626 (1995).
[430] Gabriel R. Bengochea, Gabriel León, Elias Okon, and Daniel Sudarsky, Can the quantum vacuum fluctuations really solve the cosmological constant problem?, Eur. Phys. J. C 80, 18 (2020), arXiv:1906.05406 .
[431] G. C. Wick, The Evaluation of the Collision Matrix, Phys. Rev. 80, 268–272 (1950).
[432] A.J.G. Hey and P. Walters, The New Quantum Universe (Cambridge University Press, 2003) p. 228.
[433] L.M. Brown and L. Hoddeson, The Birth of Particle Physics (Cambridge University Press, 1983) p. 56.
[434] P. A. M. Dirac, A Theory of Electrons and Protons, Proc. Roy. Soc. Lond. A 126, 360–365 (1930).
[435] Carl D. Anderson, The Apparent Existence of Easily Deflectable Positives, Science 76, 238–239 (1932).
[436] A. Denner, S. Heinemeyer, I. Puljak, D. Rebuzzi, and M. Spira, Standard Model Higgs-Boson Branching Ratios with Uncertainties, Eur. Phys. J. C 71, 1753 (2011), arXiv:1107.5909 .
[437] Julian S. Schwinger, The Theory of quantized fields. 1., Phys. Rev. 82, 914–927 (1951).
[438] Gerhart Luders, On the Equivalence of Invariance under Time Reversal and under Particle-Antiparticle Conjugation for Relativistic Field Theories, Kong. Dan. Vid. Sel. Mat. Fys. Med. 28N5, 1–17 (1954).
[439] C. S. Wu, E. Ambler, R. W. Hayward, D. D. Hoppes, and R. P. Hudson, Experimental Test of Parity Conservation in Decay, Phys. Rev. 105, 1413–1414 (1957).
[440] L. D. Landau, On the conservation laws for weak interactions, Nucl. Phys. 3, 127–131 (1957).
[441] J. H. Christenson, J. W. Cronin, V. L. Fitch, and R. Turlay, Evidence for the Decay of the Meson, Phys. Rev. Lett. 13, 138–140 (1964).
[442] Particle Data Group Collaboration, Review of particle physics, Phys. Rev. D 110, 030001 (2024).
[443] Michael Dine, Willy Fischler, and Mark Srednicki, A Simple Solution to the Strong CP Problem with a Harmless Axion, Phys. Lett. B 104, 199–202 (1981).
[444] Gerard ’t Hooft, Renormalization of Massless Yang-Mills Fields, Nucl. Phys. B 33, 173–199 (1971).
[445] Gerard ’t Hooft, Renormalizable Lagrangians for Massive Yang-Mills Fields, Nucl. Phys. B 35, 167–188 (1971).
[446] Steven Weinberg, The Quantum Theory of Fields Effective or Fundamental? (CERN, 2009) [Online; accessed 10-October-2024].
[447] L. O’Raifeartaigh, The dawning of gauge theory (Princeton Univ. Press, Princeton, NJ, USA, 1997).
[448] Michael B. Green, J. H. Schwarz, and Edward Witten, SUPERSTRING THEORY. VOL. 1: INTRODUCTION, Cambridge Monographs on Mathematical Physics (1988).
[449] Ishaan Gulrajani, Faruk Ahmed, Martin Arjovsky, Vincent Dumoulin, and Aaron Courville, Improved Training of Wasserstein GANs, in Advances in Neural Information Processing Systems 30 (Curran Associates, Inc., 2017) p. 5767, arXiv:1704.00028 .
[450] Takeru Miyato, Toshiki Kataoka, Masanori Koyama, and Yuichi Yoshida, Spectral Normalization for Generative Adversarial Networks, in 6th International Conference on Learning Representations (2018) arXiv:1802.05957 .
[451] Florian Schäfer, Hongkai Zheng, and Anima Anandkumar, Implicit competitive regularization in GANs, arXiv:1910.05852 (2019).
[452] Tero Karras, Miika Aittala, Janne Hellsten, Samuli Laine, Jaakko Lehtinen, and Timo Aila, Training Generative Adversarial Networks with Limited Data, arXiv:2006.06676 (2020).
[453] Ngoc-Trung Tran, Viet-Hung Tran, Ngoc-Bao Nguyen, Trung-Kien Nguyen, and Ngai-Man Cheung, On data augmentation for GAN training, arXiv:2006.05338 (2020).
[454] Zhengli Zhao, Zizhao Zhang, Ting Chen, Sameer Singh, and Han Zhang, Image augmentations for GAN training, arXiv:2006.02595 (2020).
[455] Martin Arjovsky, Soumith Chintala, and Léon Bottou, Wasserstein GAN, arXiv:1701.07875 (2017).
[456] Zichun Hao, Raghav Kansal, Javier Duarte, and Nadezda Chernyavskaya, Graph Neural Network Autoencoders for Jets (2022).
[457] H. G. Barrow, J. M. Tenenbaum, R. C. Bolles, and H. C. Wolf, Parametric correspondence and Chamfer matching: Two new techniques for image matching, in Proceedings of the 5th International Joint Conference on Artificial Intelligence (KJCAI), Vol. 2 (Morgan Kaufmann Publishers Inc., San Francisco, CA, USA, 1977) p. 659.
[458] Haoqiang Fan, Hao Su, and Leonidas J. Guibas, A point set generation network for 3D object reconstruction from a single image, in 2017 IEEE Conference on Computer Vision and Pattern Recognition (CVPR) (2017) p. 2463, arXiv:1612.00603 .
[459] Yan Zhang, Jonathon Hare, and Adam Prügel-Bennett, FSPool: Learning set representations with featurewise sort pooling, in 8th International Conference on Learning Representations (2020) arXiv:1906.02795 .
[460] H. W. Kuhn, The Hungarian method for the assignment problem, Naval Research Logistics Quarterly 2, 83 (1955).
[461] Raghav Kansal, Carlos Pareja, and Javier Duarte, jet-net/JetNet: v0.2.1.post2 (2022).
[462] Diederik P. Kingma and Jimmy Ba, Adam: A method for stochastic optimization, in 3rd International Conference on Learning Representations, ICLR 2015 (ICLR, San Diego, 2015) arXiv:1412.6980 .
[463] Martín Abadi et al., TensorFlow: Large-scale machine learning on heterogeneous systems (2015), software available from tensorflow.org