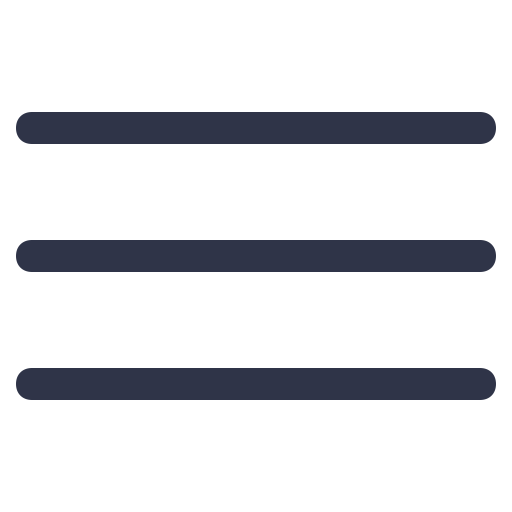
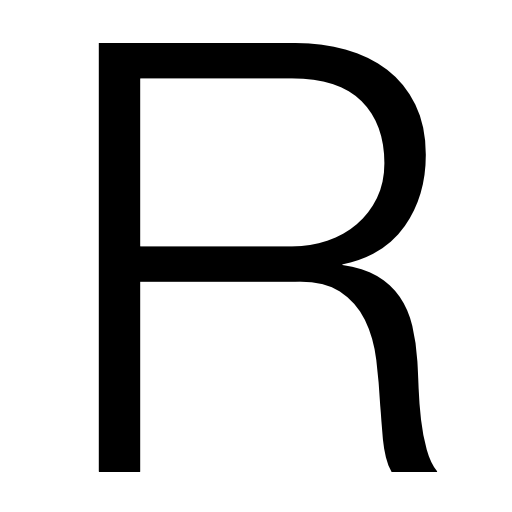
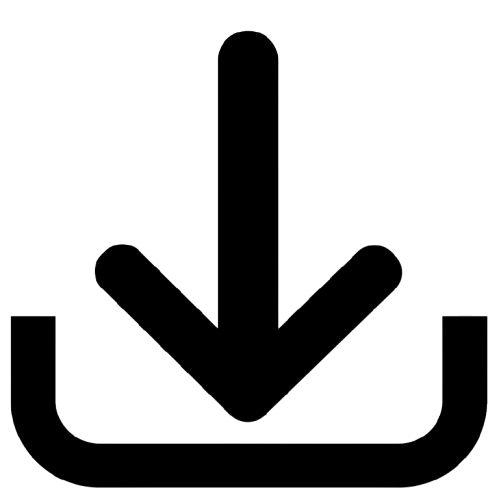
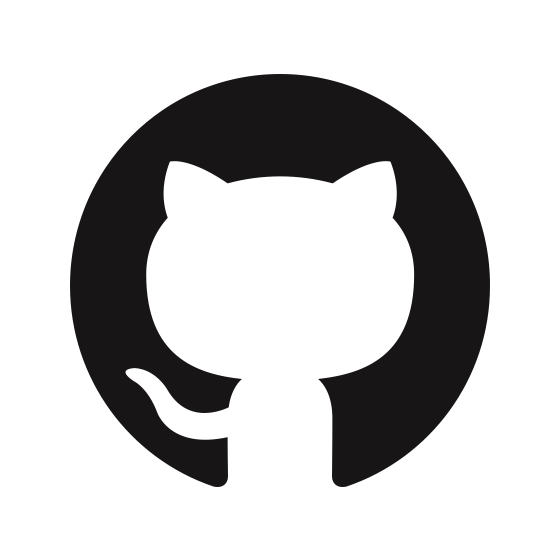
Vita
- 2019
-
Bachelor of Science in Physics and Computer Engineering
University of California San Diego
Summa cum laude - 2019
-
CERN Openlab Summer Student
- 2019
-
IRIS-HEP Fellow
- 2019-2024
-
Graduate Student Researcher
University of California San Diego - 2021-2022
-
Artificial Intelligence Fellow
Fermilab LHC Physics Center - 2023-2024
-
Graduate Scholar
Fermilab LHC Physics Center - 2024
-
Doctor of Philosophy in Physics
University of California San Diego - 2024-
-
AI/Schmidt Postdoctoral Scholar Research Associate
California Institute of Technology and Fermilab
PUBLICATIONS
Note: as a member of the CMS collaboration, I have been an author on all CMS papers
since 2019. The following includes only the CMS publications to which I made
significant contributions during my PhD.
- 1.
- CMS Collaboration, “Search for Nonresonant Pair Production of Highly Energetic Higgs Bosons Decaying to Bottom Quarks and Vector Bosons”, in prep, CMS-HIG-23-012 (2023).
- 2.
- CMS Collaboration, “Search for a massive scalar resonance decaying to a light scalar and a Higgs boson in the two b quarks and four light quarks final state”, in prep, CMS-B2G-23-007 (2023).
- 3.
- A. Li*, V. Krishnamohan*, R. Kansal, J. Duarte, R. Sen, S. Tsan, and Z. Zhang, “Induced generative adversarial particle transformers”, NeurIPS ML4PS Workshop (2023), arXiv:2312.04757.
- 4.
- R. Kansal, C. Pareja, Z. Hao, and J. Duarte, “JetNet: A Python package for accessing open datasets and benchmarking machine learning methods in high energy physics”, JOSS 8, 5789 (2023).
- 5.
- Z. Hao, R. Kansal, J. Duarte, and N. Chernyavskaya, “Lorentz group equivariant autoencoders”, Eur. Phys. J. C 83, 485 (2023), arXiv:2212.07347.
- 6.
- R. Kansal, A. Li, J. Duarte, N. Chernyavskaya, M. Pierini, B. Orzari, and T. Tomei, “Evaluating generative models in high energy physics”, Phys. Rev. D 107, 076017 (2023), arXiv:2211.10295.
- 7.
- CMS Collaboration, “Search for Nonresonant Pair Production of Highly Energetic Higgs Bosons Decaying to Bottom Quarks”, Phys. Rev. Lett. 131, 041803 (2023), arXiv:2205.06667.
- 8.
- R. Kansal, J. Duarte, H. Su, B. Orzari, T. Tomei, M. Pierini, M. Touranakou, J.-R. Vlimant, and D. Gunopulos, “Particle Cloud Generation with Message Passing Generative Adversarial Networks”, NeurIPS (2021), arXiv:2106.11535.
- 9.
- F. Mokhtar, R. Kansal, and J. Duarte, “Do graph neural networks learn traditional jet substructure?”, NeurIPS ML4PS Workshop (2022), arXiv:2211.09912.
- 10.
- M. Touranakou, N. Chernyavskaya, J. Duarte, D. Gunopulos, R. Kansal, B. Orzari, M. Pierini, T. Tomei, and J.-R. Vlimant, “Particle-based fast jet simulation at the LHC with variational autoencoders”, Machine Learning: Science and Technology 3, 035003 (2022), arXiv:2203.00520.
- 11.
- A. Apresyan, D. Diaz, J. Duarte, S. Ganguly, R. Kansal, N. Lu, C. M. Suarez, S. Mukherjee, C. Peña, B. Sheldon, and S. Xie, “Improving Di-Higgs Sensitivity at Future Colliders in Hadronic Final States with Machine Learning”, Contribution to Snowmass Summer Study (2022), arXiv:2203.07353.
- 12.
- Y. Chen et al., “A FAIR and AI-ready Higgs boson decay dataset”, Sci. Data 9, 31 (2021), arXiv:2108.02214.
- 13.
- F. Mokhtar, R. Kansal, D. Diaz, J. Duarte, J. Pata, M. Pierini, and J.-R. Vlimant, “Explaining machine-learned particle-flow reconstruction”, NeurIPS ML4PS Workshop (2021), arXiv:2111.12840.
- 14.
- S. Tsan, R. Kansal, A. Aportela, D. Diaz, J. Duarte, S. Krishna, F. Mokhtar, J.-R. Vlimant, and M. Pierini, “Particle graph autoencoders and differentiable, learned energy mover’s distance”, NeurIPS ML4PS Workshop (2021), arXiv:2111.12849.
- 15.
- B. Orzari, T. Tomei, M. Pierini, M. Touranakou, J. Duarte, R. Kansal, J.-R. Vlimant, and D. Gunopulos, “Sparse Data Generation for Particle-Based Simulation of Hadronic Jets in the LHC”, ICML LXAI Workshop (2021), arXiv:2109.15197.
- 16.
- R. Kansal, J. Duarte, B. Orzari, T. Tomei, M. Pierini, M. Touranakou, J.-R. Vlimant, and D. Gunopulos, “Graph generative adversarial networks for sparse data generation in high energy physics”, NeurIPS ML4PS Workshop (2020), arXiv:2012.00173.