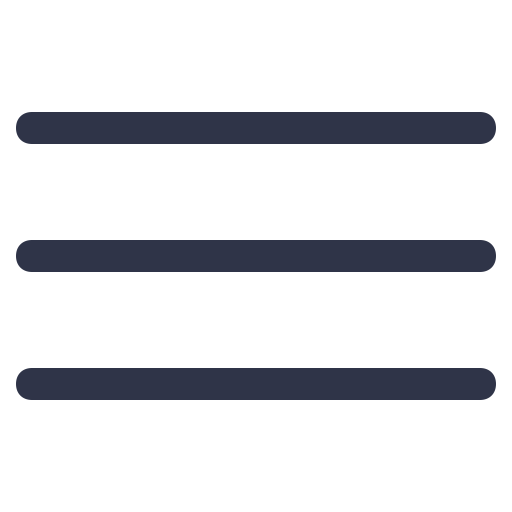
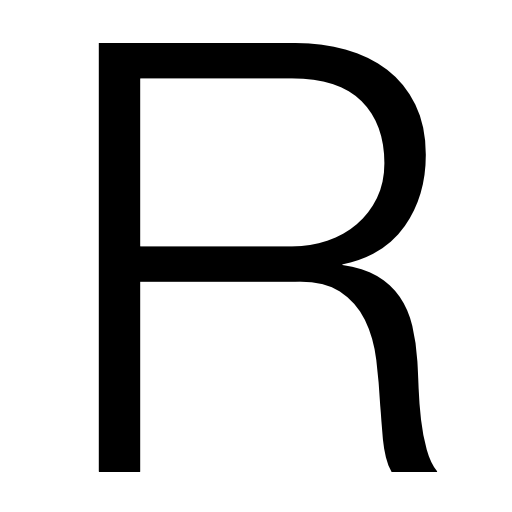
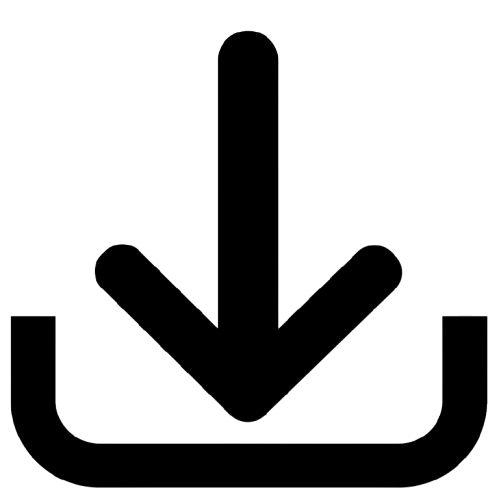
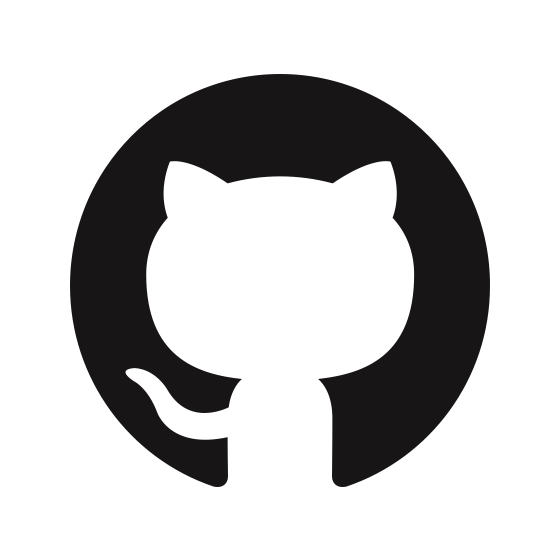
Chapter 12
Conclusion and impact
In this Part, we argued for the necessity of a new generation of fast and accurate simulators in HEP to meet the computational demands of Run 3 and HL-LHC. To this end, we introduced two state-of-the-art (SOTA) ML-based generative models, MPGAN and iGAPT, which leverage the success of generative adversarial networks (GANs) in computer vision, but incorporate critical physical inductive biases of our data to achieve breakthrough performance in simulating high-energy jets. Specifically, they use permutation invariant graph- and attention-based architectures, respectively, to operate on point cloud representations of jets and model the interactions of their particle constituents and global correlations. In the case of iGAPT, we further introduce the novel “induced particle attention blocks” which offer linear time complexity and high computational efficiency by building physics insight into the attention mechanism. Both models are able to model well physical observables of jets and, crucially, generate jets at orders of magnitude faster than traditional simulators.
We also discussed the critical challenge of validating such models, to ensure trust in their applications in HEP. Several methods were reviewed and systematically evaluated, and the novel Fréchet and kernel physics distances (FPD and KPD) were introduced as efficient, interpretable, and highly sensitive metrics for evaluating generative models in HEP. Their performance, along with Wasserstein () distances between individual features and the neural-network-based Fréchet ParticleNet distance (FPND), was demonstrated on toy distributions as well as on evaluating the MPGAN and iGAPT models. We propose FPD, KPD, and distances as effective metrics for evaluating fast simulators, and recommend their adoption by CMS and other collaborations in validating the next generation of simulators.
This work has proven impactful in the HEP community, leading to several follow-up studies on point-cloud generative modeling in HEP using the JetNet dataset and our proposed evaluation metrics, benchmarked against MPGAN and iGAPT [375–385]. JetNet and our metrics have been used to develop and benchmark a variety of novel architectures such as diffusion and conditional flow-matching models [379, 384–386], foundation models [387], equivariant models [68] and even quantum GNNs [388] on myriad applications including calorimeter and future detector simulations [389, 390], anomaly detection, and jet classification. Ongoing work explores the adoption of our metrics in the CMS release validation pipeline and our models for CMS fast simulation.