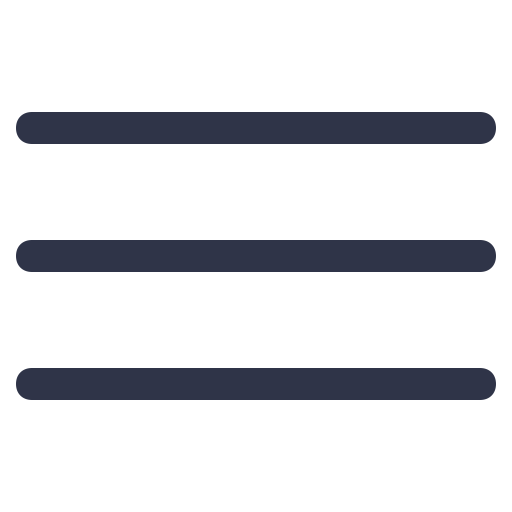
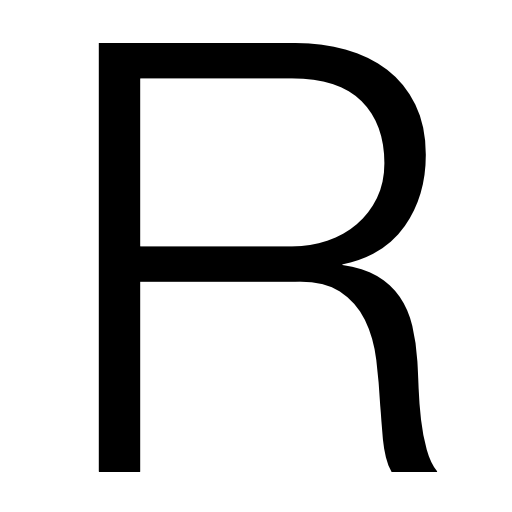
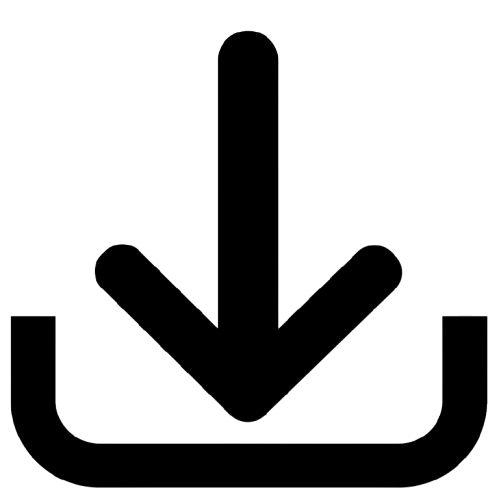
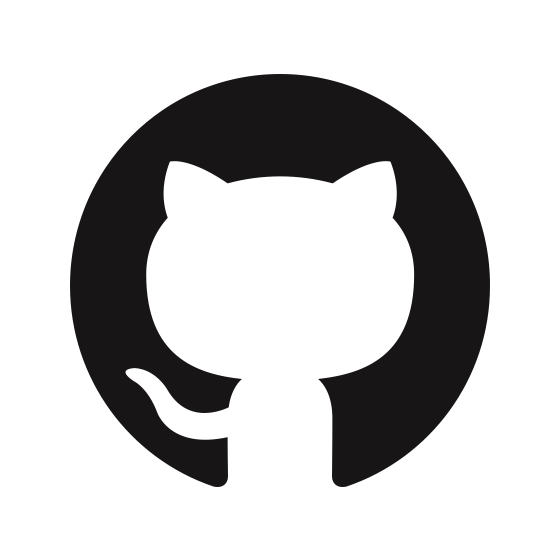
11.5 Summary
We discussed several potential evaluation metrics for generative models in HEP, using the framework of two-sample GOF testing between real and simulated data. Inspired by the validation of simulations in both physics and machine learning, we introduce two new metrics, the Fréchet and kernel physics distances, which employ hand-engineered physical features, to compare and evaluate alternative simulators. Practically, these metrics are efficient, reproducible, and easily standardized, and, being multivariate, can be naturally extended to conditional generation.
We performed a variety of experiments using the proposed metrics on toy Gaussian-distributed and high energy jet data. We illustrated as well the power of these metrics to discern between state-of-the-art ML models for simulating jets: MPGAN and iGAPT. We find that FPD is extremely sensitive to expected distortions from ML generative models, and collectively, FPD, KPD and the Wasserstein 1-distance () between individual feature distributions, should successfully cover all relevant alternative generated distributions. Hence, we recommend the adoption of these metrics in HEP for evaluating generative models. Future work may explore the specific set of physical features for jets, calorimeter showers, and beyond, to use for FPD and KPD.
Acknowledgements
This chapter is, in part, a reprint of the materials as they appear in Phys. Rev. D, 2023, R. Kansal; A. Li; J. Duarte; N. Chernyavskaya; M. Pierini; B. Orzari; and T. Tomei; Evaluating generative models in high energy physics. The dissertation author was the primary investigator and author of this paper.