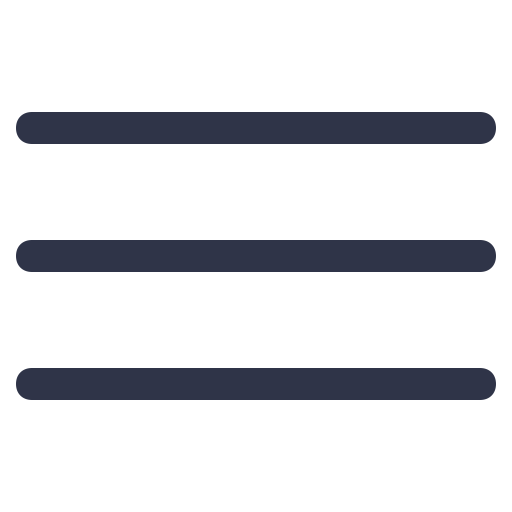
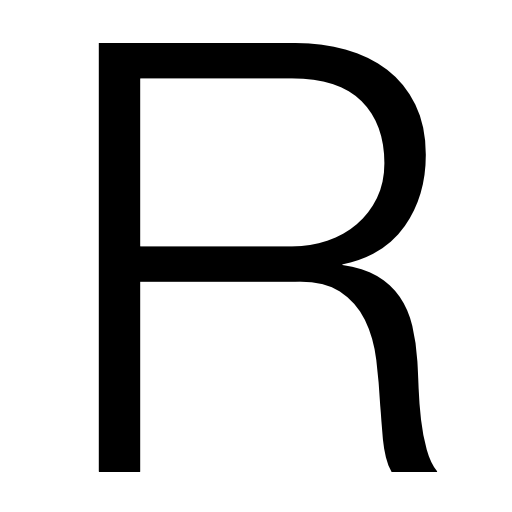
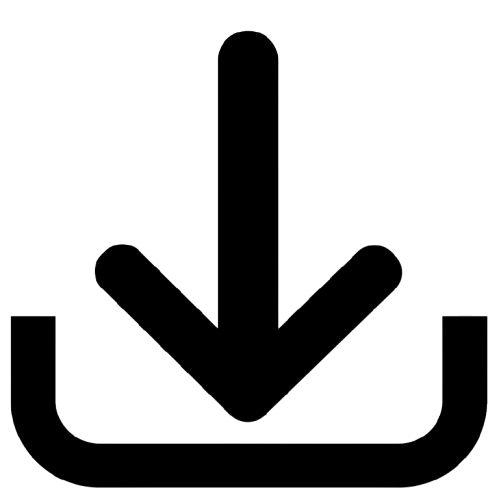
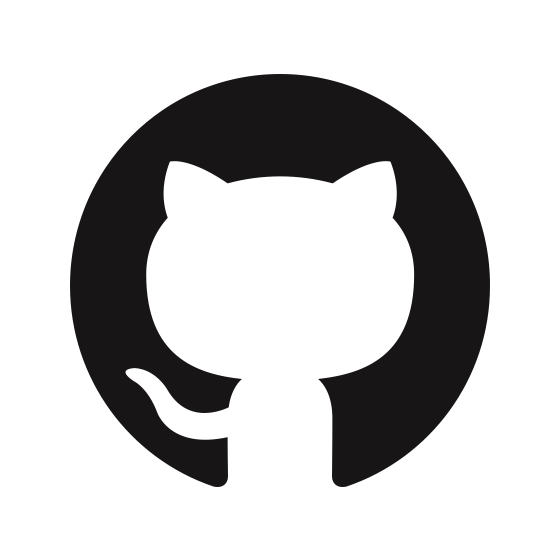
16.4 Conclusion
We develop the Lorentz group autoencoder (LGAE), an autoencoder model equivariant to Lorentz transformations. We argue that incorporating this key inductive bias of high energy physics (HEP) data can have a significant impact on the performance, efficiency, and interpretability of machine learning models in HEP. We apply the LGAE to tasks of compression and reconstruction of input quantum chromodynamics (QCD) jets, and of identifying anomalous top quark, W boson, and Z boson jets. We report excellent performance in comparison to baseline graph and convolutional neural network autoencoder models, with the LGAE outperforming them on several key metrics. We also demonstrate the LGAE’s interpretability, by analyzing the latent spaces of LGAE models for both tasks, and data efficiency relative to baseline models. The LGAE opens many promising avenues in terms of both performance and model interpretability, with the exploration of new datasets, the magnitude of Lorentz and permutation symmetry breaking due to detector effects, higher-order Lorentz group representations, and challenges with real-life compression and anomaly detection applications all exciting possibilities for future work.
Acknowledgements
This chapter is, in part, a reprint of the materials as they appear in Eur. Phys. J. C, 2023, Z. Hao; R. Kansal; J. Duarte; and N. Chernyavskaya; Lorentz group equivariant autoencoders. The dissertation author was the primary investigator and (co-)author of this paper.