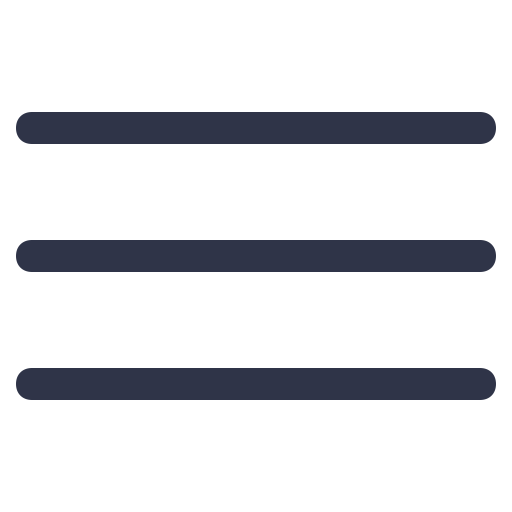
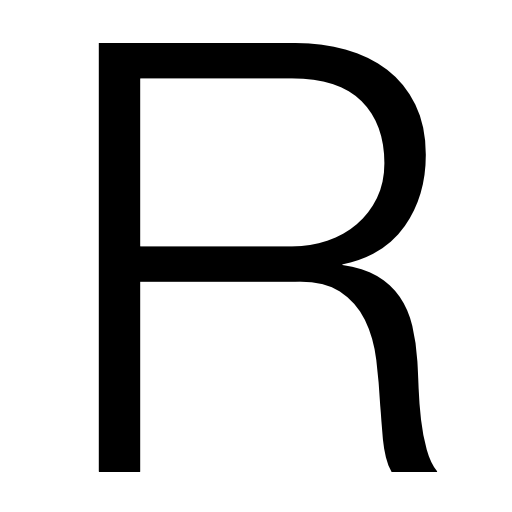
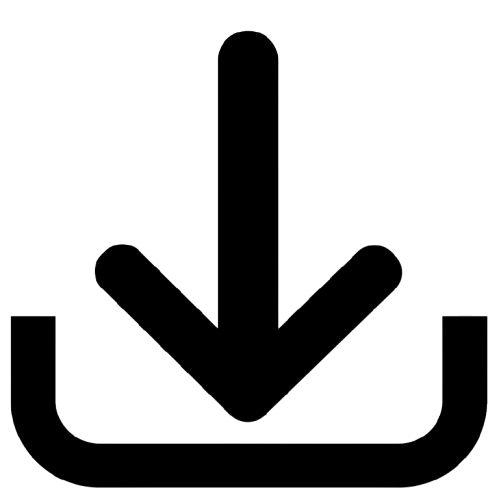
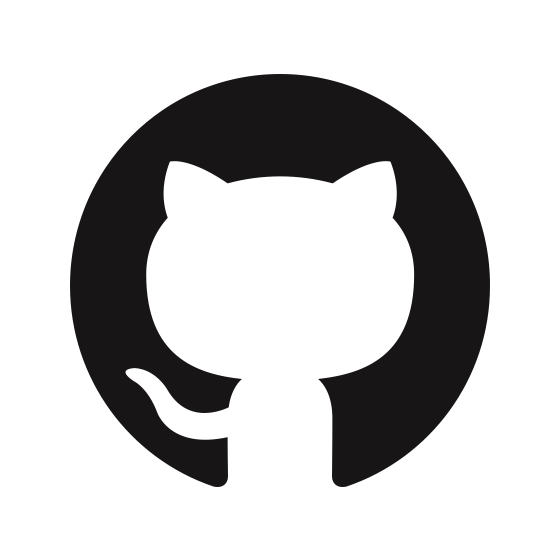
Chapter 13
Boosted Higgs identification
In Part V, we describe searches for the nonresonant SM and resonant BSM Higgs boson pair production, in the decay channel. As discussed in Chapter 4.3, classifying the merged and jets is a key component of these analyses. Traditionally, jet-tagging involved hand-engineering high-level features, such as the jet mass, substructure variables, and secondary vertices, to manually define selections for such jets. The advent of deep learning techniques, which are able to make effective use of low-level particle and vertex data, however, has revolutionized this process.
This is epitomized by the ParticleNet tagger [230], described in Section 13.1, which uses a graph neural network (GNN) architecture to model individual jet constituents and their interactions, and has demonstrated strong performance on tagging (among other final states). However, a similarly high performing classifier has not yet been developed for four-pronged jets. In this chapter, we showcase the development of a new attention-based ParticleTransformer model [391] to identify such boosted jets, which has proven to be a critical component of not only this analysis, but many ongoing boosted Higgs searches in CMS. We additionally present a novel calibration method for the tagger, which improves the systematic uncertainties on signal efficiency scale factors from 50% using a previous proxy-based method [392] down to 16%, representing a significant improvement in analysis sensitivity.