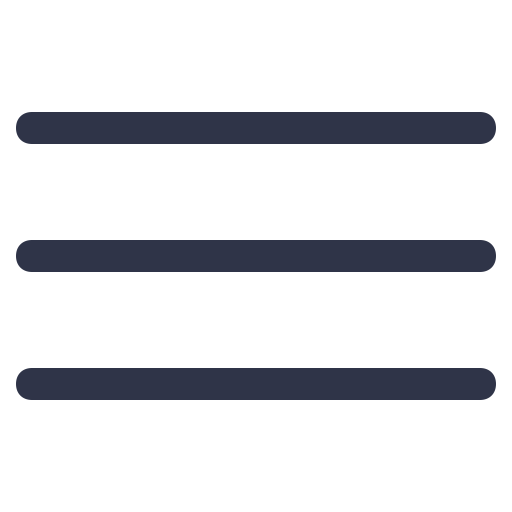
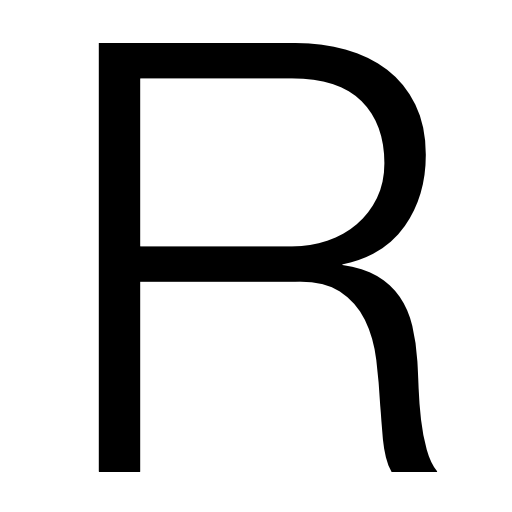
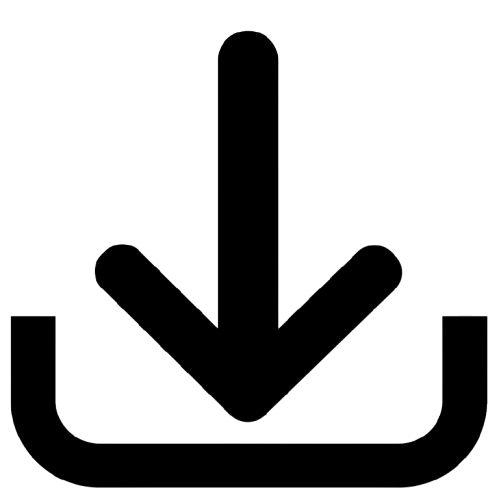
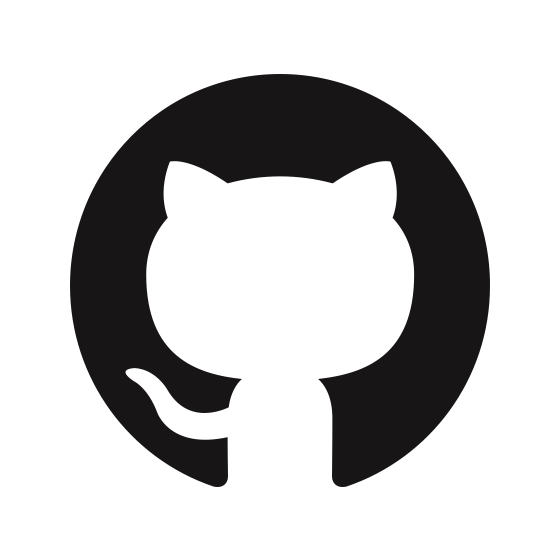
10.2 Generative adversarial particle transformers
In the previous section, we introduced the MPGAN model, which represented a significant advance in ML-based fast simulations for HEP, being able to capture the complex global structure of jets and handle variable-sized particle clouds using fully-connected graph neural networks. However, this fully-connected nature means its memory and time complexity scale quadratically with the number of particles per jet, leading to difficulty in simulating larger particle clouds.
In this section, we first introduce in Section 10.2.1 the generative adversarial particle transformer (GAPT) model, which takes advantage of the computationally efficient “attention”-mechanism that has led to significant advances in natural language processing. This provides a significant speed-up over MPGAN, but is still limited by the quadratic scaling of the attention mechanism. We then present the induced GAPT (iGAPT) model in Section 10.2.2, featuring “induced particle attention blocks” (IPABs) that incorporate physics-informed inductive biases of jets, to offer both linear-time complexity and improved output fidelity. We discuss architecture choices and timing comparisons, but defer a deeper evaluation of the performance of these models and MPGAN to Chapter 11, which details our new methodology for quantitatively validating and comparing fast simulations.
10.2.1 GAPT
Similar to MPGAN, GAPT is a GAN for particle cloud data, but employing self-attention instead of message passing in the two generator and discriminator networks. It is based on the generative adversarial set transformer (GAST) architecture [333], which makes use of set transformer [334] blocks to aggregate information across all points and update their features. It maintains the key inductive biases which makes MPGAN successful—permutation symmetry-respecting operations, and fully connected interaction between nodes during generation to learn high-level global features, but with a significant improvement in speed due to the higher computational efficiency of the attention mechanism compared to the message passing operations used in MPGAN.
The generator and discriminator networks are composed of permutation-invariant multihead self-attention blocks (SABs) as defined in Ref. [334] and illustrated in Figure 7.6. We use four and two SAB blocks in the generator and discriminator respectively. Each SAB block uses 8 attention heads, and a 128-dimensional embedding space for each of the query, key, and value vectors. It also contains a one-layer feed-forward neural network (FFN) after each attention step, which maintains the 128-dimensional embedding for node features, and applies a leaky ReLU activation, with negative slope coefficient 0.2. Residual connections to the pre-SAB node features are used after both the attention step and FFN. After the final SAB block, a tanh activation is applied to the generator, whereas in the discriminator, the results are first pooled using a pooling by multihead attention (PMA) block [334], followed by a final fully connected layer and sigmoid activation.
For training, we use the mean squared error loss function, as in the LSGAN [335], and the RMSProp optimizer with a two timescale update rule [324], using a learning rate of and for the discriminator and generator respectively. Dropout, with probability 0.5, is used to regularize the discriminator. We train for 2000 epochs and select the model with the lowest Fréchet physics distance.
10.2.2 iGAPT
The iGAPT model builds on the GAPT architecture, but introduces a novel physics-informed attention mechanism that allows for linear-time complexity in the number of particles per jet. As illustrated in Figure 10.8, it is a GAN with the generator and discriminator networks composed of “induced particle attention blocks” (IPABs). On top of maintaining permutation invariance and operating on point-cloud representations, as in MPGAN and GAPT, the key inductive bias we experiment with in iGAPT is maintaining a global vector through the generation and discrimination processes, , which implicitly represents global jet features. IPABs and different ways of incorporating into the attention process are described below.
The generation process starts with sampling random Gaussian noise and a particle multiplicity from the true distribution, which is transformed via a learned linear embedding layer. The noise has two components: a set of vectors representing initial particle features, and a single vector representing initial jet features. is the maximum number of particles per jet we want to simulate, and the number of initial particle and jet features is a hyperparameter we tune. The jet noise is added to the embedded to produce , which is then transformed along with the particle noise via multiple IPABs to output a generated jet.
The discrimination process starts with a generated or real jet, and the sampled or true jet multiplicity . This is again transformed via a learned embedding layer to produce the conditioning vector for the discriminator, which along with the input jet are processed through IPABs producing an intermediate jet representation. The constituents of this jet are aggregated in a permutation-invariant manner using a pooling by multihead attention (PMA) layer, as introduced in [334], the output of which is finally fed into a linear layer to classify the jet as real or fake.
Attention blocks require fixed multiplicity; however, jets naturally have a variable number of particle constituents. To handle this, we zero-pad all jets to particles and use masked attention in every block to ignore these.
Attention Blocks
The SAB blocks used by GAPT offer the benefit of global, fully connected interactions between particles; however, this also results in quadratic scaling with the particle multiplicity, which is undesirable. To counter this, Ref. [334] also proposed “induced” SABs (ISABs), where the input set is first attended to by learned inducing vectors via a MAB, outputting an intermediate, compressed representation. This representation is then attended to by the original set to produce the new output. This retains the global interactions between particles while achieving scaling—linear in the particle multiplicity. ISABs have been used in generative adversarial set transformers (GAST) [333], yielding high quality results on computer vision datasets. GAST incorporates similar global set features by concatenating them to each particle before every ISAB operation.
In iGAPT, we experiment with an alternative version of conditioning, which we call “induced particle attention”. Here, is directly used as the inducing vector in an ISAB, and is continuously updated through the intermediate, induced attention outputs. Explicitly, as illustrated in Figure 10.9, the th IPAB receives as input the jet and global features from the previous block, after which is first attended to by to output updated features , and then conversely is attended to by to output the updated jet . This is interpreted as a way to update and learn the global jet features, such as mass and , along with the individual particle features, and allow both to interact in each attention layer. An additional and significant advantage is that this induced attention operation involves only one inducing vector — , hence and we achieve computational complexity.
Training and Hyperparameters
Along with GAPT, we test both the GAST and iGAPT models on the JetNet dataset, to compare the performance of ISABs and IPABs. The iGAPT and GAST models were trained for a maximum of 6000 epochs on a single NVIDIA RTX1080 GPU using the RMSProp optimizer. The training time and batch size for 30- and 150-particle gluon jets is shown in Table 10.3. We use a two-time update rule [324], with learning rates of and for the generator and discriminator, respectively. The discriminator is regularized with dropout with a probability of 0.5 and layer normalization. We use a LeakyReLU activation after every linear layer, except the final generator (tanh) and discriminator (sigmoid) outputs. After hyperparameter tuning, we settle on on 3 and 6 ISAB layers for the generator and discriminator respectively, and 4 and 8 IPAB layers for the iGAPT model. We use 16 and 128 initial particle and jet features, respectively, in iGAPT. GAST uses 20 inducing vectors for its ISABs.
10.2.3 Experiments
Results
We test and compare the GAPT, GAST, iGAPT, and MPGAN models on 30-particle gluon, light quark, and top quark jets, and on 150-particle gluon jets. Out of all our trainings, we select the GAPT, GAST, and iGAPT models with the lowest Fréchet physics distance score, as will be introduced in Section 11, due to its high sensitivity to common types of mismodeling. Comparisons of real and iGAPT- and MPGAN-generated feature distributions are shown in Figure 10.10 and Appendix C.2.1 for 30 and 150 particles, respectively, demonstrating high fidelity results from both models. We defer a detailed evaluation of the performance to Section 11.
Timing
A key benefit of iGAPT is its improved time complexity over MPGAN. This is demonstrated in Table 10.3, which shows the training and generation times for each model for 30 particle jets using the largest batch size possible on an NVIDIA 1080 GPU, with iGAPT outperforming MPGAN by a factor of 3.5. MPGAN is computationally challenging to extend to 150 particles, hence timing information is not provided; in contrast, iGAPT’s training and generation times scale well with the number of particles. Finally, we note that the “true” generation time per jet is approximately 50 ms (see Section 10.1.4.), thus iGAPT represents more than a factor of 100 speed up.
10.2.4 Summary
We introduced the attention-based generative adversarial particle transformer (GAPT) and induced GAPT (iGAPT) models for fast simulation of particle clouds, and demonstrated their performance visually on the JetNet dataset. The iGAPT model, in particular with its induced particle attention blocks (IPABs), offers a significant improvement in time complexity over MPGAN, with promising potential to scale up to the cardinality necessary for (HL-)LHC simulations.
As seen from Figure 10.10, while visually we can observe roughly that the iGAPT and MPGAN models perform similarly and match the real distributions, this is not sufficient to draw robust and objective conclusions about the models. In the next chapter, we tackle the problem of quantitatively evaluating and comparing such fast simulators in HEP.
Acknowledgements
This chapter is, in part, a reprint of the materials as they appear in the NeurIPS ML4PS Workshop, 2020, R. Kansal; J. Duarte; B. Orzari; T. Tomei; M. Pierini; M. Touranakou; J.-R. Vlimant; and D. Gunopulos. Graph generative adversarial networks for sparse data generation in high energy physics; NeurIPS, 2021, R. Kansal; J. Duarte; H. Su; B. Orzari; T. Tomei; M. Pierini; M. Touranakou; J.-R. Vlimant; and D. Gunopulos. Particle Cloud Generation with Message Passing Generative Adversarial Networks; and the NeurIPS ML4PS Workshop, 2024, A. Li; V. Krishnamohan; R. Kansal; J. Duarte; R. Sen; S. Tsan; and Z. Zhang; Induced generative adversarial particle transformers. The dissertation author was the primary investigator and (co-)author of these papers.